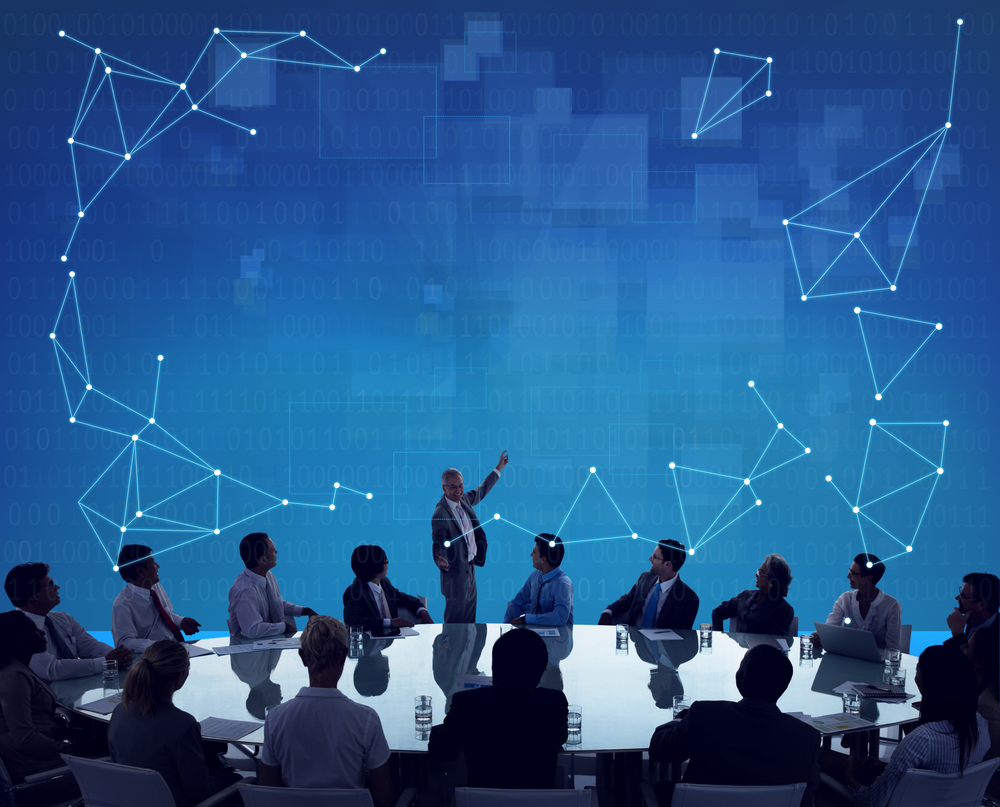
The pressure of modern data delivery to meet higher volumes, variety, and velocity of data has been turned up to 10 in the past two years, with the pandemic supercharging many industries data collection to better inform users and improve applications. Many organizations are attempting to collect more data with the same tools as previous generations and are not taking advantage of new processes such as data observability.
Without observability, an organization cannot be fully aware of broken pipelines, poor data quality, or cost-to-value. With it, organizations can study the health of enterprise data environments, apply machine learning to familiar methodologies for data quality, optimize data delivery across distributed architectures, and contribute to DataOps initiatives.
Data observability is part of a larger landscape of observability. With data observability, there are two disciplines of focus: data quality and data pipeline. Data quality observes the accuracy, completeness, and consistency of data, while data pipeline looks at resource performance, availability, and cost.
SEE ALSO: Making the Case for a Small Data Observability Strategy
There are three lifecycle stages for data observability. The first is validation and detection, in which the program detects patterns, anomalies, outliers, and other nodes of data. From there, the observability platform should make assessments and predictions, which can be in the form of measuring impact, correlating events, or isolating root causes. Once assessments have been made, the data can then be used to resolve issues or prevent future events from happening. “Your number one goal is to prevent issues affecting customers. That involves fast resolution and proactive identification,” said Kevin Petrie, VP of research at Eckerson Group at the CDO TechVent virtual event on Data Observability.
Some of the key success factors are establishing a strategic plan at the start of the project, with a capable data leader who is able to build a cross-functioning team to build the data observability program. The team must identify key control points within the data pipeline that make the most sense to check quality and risk. This can reduce bottlenecks and other issues with data quality and data pipeline checks in the future.
See also: Observability: Key to Managing Complex Infrastructures
“There’s a lot of enthusiasm about tools, about the ways in which anomaly detection machine learning algorithms are helping to adapt to this new world where you have cloud-driven, digital transformation-driven environments which need a lot more to track,” said Petrie. “But, you also need people and process and that’s an overriding factor as a success factor in data observability.”
Read the rest of this article on RTInsights.
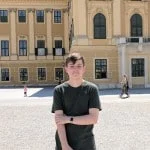