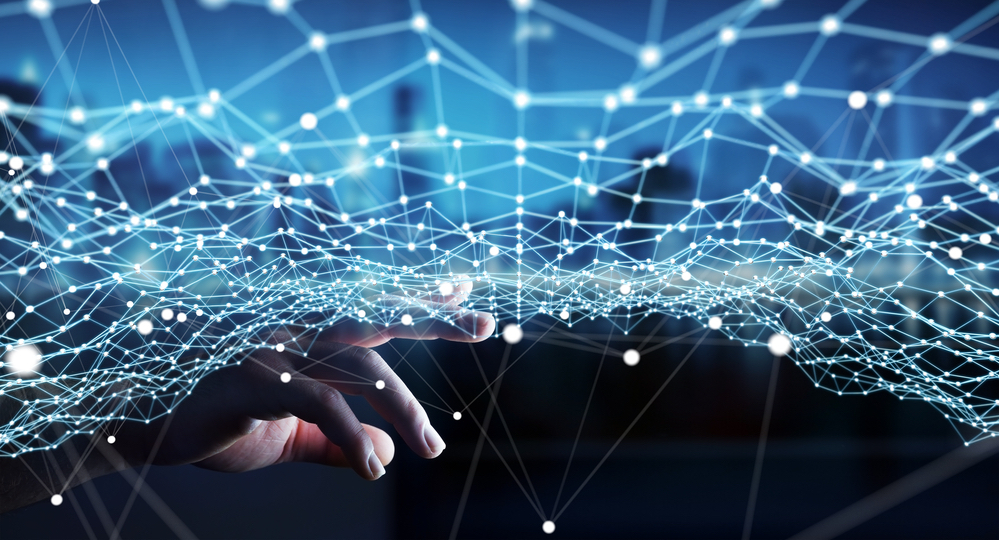
The digital transformation brought about by the post-pandemic era has significantly impacted customer needs and attitudes. As companies strive to navigate these changes, data has emerged as a crucial tool for identifying areas of improvement, increasing profitability, and accelerating product innovation. CDInsights.ai sits down with Satyen Sangani, CEO and co-founder of Alation, to talk about the technical challenges posed by complex data landscapes, and how companies can leverage emerging technologies and trends to thrive in the digital age.
How have your customers’ needs or attitudes changed given current economic conditions and post-pandemic digital transformation?
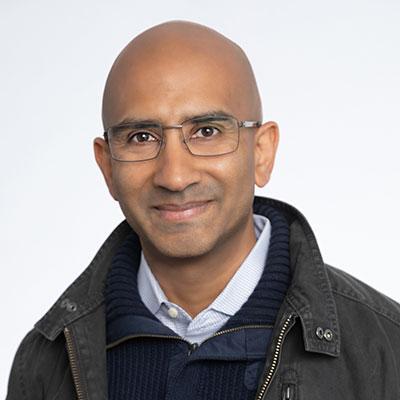
Satyen: A challenging economic environment requires organizations to operate efficiently. Data helps companies identify areas where they can become more efficient and measure their improvement. We see many of our customers use data to increase profitability or bring new products to market faster while improving operations. For example, despite a couple of turbulent years during the pandemic, Alation customer Airlines Reporting Corporation (ARC) now brings new products to market faster by making data more accessible across the business.
Those that will emerge as winners take a data-centric approach to business, regardless of the economic conditions, which enables an organization to be more transparent, flexible, and efficient.
How are you responding? Are you conveying your offerings’ value differently? Have you made or planned adjustments in your product strategy or roadmap?
Satyen: Our customers rarely, if ever, chase technology for the sake of technology. They’ve always sought to derive business value from technology through increased productivity, risk mitigation, and cost optimization. Current macroeconomic headwinds have only accelerated sensitivity to value-based messaging and, as a result, we continue to emphasize outcomes, value, and customer proof points across our messaging and range of use cases we address as the leading data intelligence platform, including data governance, self-service analytics, and cloud transformation.
Alation’s value is a function of how well we help customers manage the complexity of their environment and how widely the platform is adopted across stakeholders. Our product strategy reflects this as we’ve accelerated our investments in open metadata initiatives, including the Open Connector Framework and Open Data Quality Initiative. Customers don’t want us to do everything – they want us to connect to everything to help them make sense of all metadata and, ultimately, put the right data in the right hands at the right time. Additionally, our investments in Alation Anywhere (Alation Connected Sheets, Slack, and Microsoft Teams) help get the right data into everyone’s hands, not just data professionals.
What are the main technical challenges your customers face? Does this mark a change from a year ago?
Satyen: The leading challenge our customers face is an increasingly complex data landscape, with data that spans applications, databases, data warehouses, hybrid cloud environments, and more. As the modern data stack grows in complexity and organizations deploy an increasing array of disparate data sources across on-prem, cloud, and hybrid environments, enhancing visibility across the data stack is critical to improving data trust. And every year, this complexity will only worsen as organizations make headway on digital transformation. We simplify this complexity by being the most extensible data intelligence platform on the market, allowing our customers to extend visibility to a greater number of BI platforms, databases, data transformation tools, and cloud apps for a more holistic view of their data.
Which emerging technology or business trend do you think will have the most impact on your company or your customers?
Satyen: The market for data fabric and data mesh has advanced from even a few months ago. Organizations are now wondering which vendors support elements of the data fabric stack. And they’re wondering how to implement some principles of data mesh (i.e., the market is going deeper and expecting value from their architectures instead of pursuing tech for the sake of tech).
The two architectural approaches work well together to fulfill similar objectives – this is because many organizations leveraging data analytics rely on shared data to make good decisions. However, data is widely (and elaborately) distributed across an organization, and these data landscapes are complex and challenging to navigate. Data fabric enables friction-less access and sharing of data – a key trend that simplifies complex data infrastructures and supports metadata-driven use cases across hybrid and multi-cloud environments.
Data mesh, too, is essential. Think of it as a self-serve data platform that empowers teams to autonomously own their data products and deliver them to consumers. It inverts the traditional enterprise data supply chain to decentralize data flow. So, instead of having a central team manage all data for the company, data mesh democratizes responsibilities to ensure high-quality data is managed by domain experts, who are best equipped to deliver it to data consumers faster and more efficiently. This enables organizations to scale their data systems in a more manageable way.
CDI: More important than choosing a Data Fabric or a Data Mesh approach is investing time in the data catalog as that’s what is going to enable the kind of data product delivery acceleration that ARC experienced. Can you tell us more about the data catalog’s role in migrating to the cloud?
Satyen: A data catalog means a lot of things to a lot of different people. It might seem like arcane technology, but– at least in how we sell the catalog – the experience is exactly the opposite. And I think it’s how the catalog is implemented. The thing that primes the pump for us is this notion of inspecting the query logs. The inspection of those query logs allows you to build a fully populated catalog that doesn’t require all of the brute force manual effort that other catalogs require. And what that means is that in data migration, you can know which on-premise workloads are actually being leveraged and used and which ones are not. You can know which data sets are being used and which ones are not.
The consequence of that is that you know what data to migrate sooner and faster and you know what data people are using. That ability to fuel discovery more efficiently is what really powers great downstream utilization and consumption, which is what ultimately allows them to build these better data products.
CDI: Is there AI involved in that ability to translate query log information into catalog information?
Satyen: There is some [AI being used] because often what you find in the logs is a lot of truncated names. So a lot of what we have to do is extrapolate those entities into something that’s more human comprehensible. We are using some version of generative AI in order to be able to do that work and allow [that data] to be searchable by people.
CDI: In the early days of AI hype, there was a lot hand waving claims. I think it’s important for vendors to talk in more detail about how their product leverages AI and to what degree the human is still included in the loop. Could you share your thoughts on that?
Satyen: Obviously, new advances make it really hard to disambiguate machine generated content from human generated content. I think that the way forward, unfortunately, is that the tools that we develop are going to have to be sophisticated enough to help humans recognize what is valid and what is deceitful. This combination of people and content is important because at the cutting edge, it’s human judgment that moves us forward and allows us to discern from whatever was known in the past.
CDI: What you’re emphasizing is that it’s not the quantity of intelligence. It’s the quality, that human intelligence and artificial intelligence are qualitatively different. The human contribution is judgment. You’re laying out a more nuanced version of this clash of concepts.
Satyen: I think it’s our job as software vendors to basically introduce that in the flow of the software. So where should people exercise judgment? How is it that you know? We don’t want to take over the judgment for people. We want to introduce it at the right moment so that people can leverage the compute in a way that’s most powerful and relevant to them.
CDI: Have you had any customers that are worrying about the legal or social justice implications of using software that doesn’t give that gap where the human can enter into the process?
Satyen: We have not yet. I think we’re still in a place where we’re doing a lot of “data janitorial” work. A lot of that work is stretched translation, and tends to be fairly bias free. But I can imagine a world in which over the next three to five years we do get to work that’s more positive, and therefore have to introduce a little bit more transparency into the decisions that we’re making on behalf of the user.
But I would imagine that systems – obviously, anything to do with people in HR, anything to do with marketing and recommendations – are the ones that are going to be at the forefront because those are the ones where discrimination and bias are probably the most prevalent and impactful to people’s decisions.
The real challenge with these tools is nobody really adopts them. And the bigger challenge is that it’s not that the tools don’t exist and people don’t do these things in governance and lineage and cataloging. It’s that a company doesn’t show what is not available to people. That said, the adoption rates are really low and I think that’s where we focus a lot of our time, energy and effort because that’s what leads to customer success much more broadly. Getting value out of data is what people are struggling to do.
If you look at this downturn what seems to be happening is a reset in people’s level of investment in data because data is an optional investment. Lots of companies are examining how much value they’re getting out of their data estate. I think we software providers have a real role to play in quantifying that for people.
CDI: What do you do that prevents your product from being shelfware but actually used to derive value for organizations?
Satyen: Our pricing model absolutely is based on utilization, with a certain baseline price of entry. But scaling is based on the level of usage in the platform. And that’s purposeful because we want people to be delivering dollars to us when they’re realizing dollars from their data. And if those two things aren’t broadly correlated, they’re going to question the value of their investment. Ultimately you can do a lot with data and get zero value. Or you can do very little and get some massive value–so knowing where and when and how to invest is a big thing.
—
Bio: Satyen Sangani is the CEO of Alation and a co-founder. In founding Alation, he aspired to help people dispassionately observe the world around them, empowering them to passionately work to improve it. Before Alation, Satyen spent nearly a decade at Oracle, where he ran the Financial Services Warehousing and Performance Management business. Prior to Oracle, Satyen was an associate with the private investment firm, Texas Pacific Group and an analyst with Morgan Stanley & Co. He holds an MS in economics from the University of Oxford and a Bachelor’s from Columbia University.
Elisabeth Strenger is a Senior Technology Writer at CDInsights.ai.