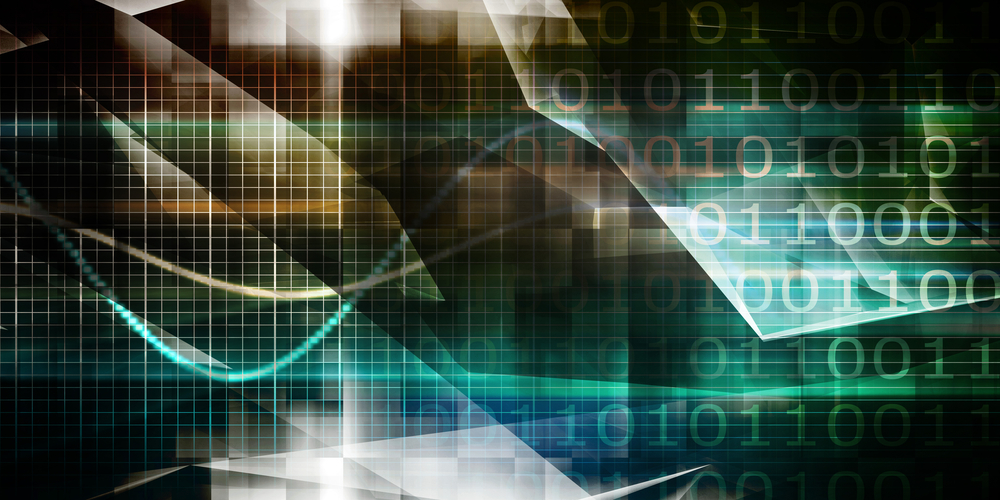
Cloud Data Insights (CDI) had the opportunity to talk with Yang Li, Co-founder and CTO of Kyligence and co-creator of the open-source OLAP project, Apache Kylin, at the Gartner Data and Analytics Summit in August. Yang Li described how he bridges the business context of data utilization and the data practitioner’s technical understanding.
(The interview has been lightly revised for clarity and readability.)
CDI: I saw that Kyligence emphasizes that their platform is an intelligent OLAP platform. Can you explain what you mean by that? Where does the intelligence come from and how does it benefit the user?
Yang Li: Let me provide some context first. The platform is designed to create a balance between data governance and data innovation. The business side of an organization thinks of data in terms of metrics, so we describe our platform to them as a metrics store, but it’s really an advanced version of a unified semantic layer. On the technical side, the discussion is about data ingestion from transactional systems, logs, securities, and, most important–multidimensional models from which the metrics are built. These two perspectives must be unified during the first phase of data governance so that there is a common data language defined right across the whole company, hopefully, such that when we say revenue in a business meeting, everybody is pointing to the same number. Otherwise, they’ll talk about the meaning of each number instead of how to improve them. That’s the real value of governance.
CDI: Having a common language certainly facilitates understanding across roles–it’s a higher-order definition of semantics, a term that can seem overly technical to all but data professionals and linguists.
Yang Li: Business customers don’t want to hear about multidimensional data layers or governance–they just hear complexity and slowdowns. The metrics store is basically another way to express a data model in business terms.
CDI: We heard during today’s keynote from Debra Logan, Distinguished VP Analyst with Gartner, that “Governance” is a scary term. Can you tell us more about the balance between governance and data innovation?
Yang Li: The business side is starting to see the value of a governed, centralized definition of metrics. In that first phase of governance, we end up with a set of governed or base metrics. Once we have established those, we want to add some innovation on top. That’s what enables business people to use data themselves. We can think of this balance as a governed, business-enriched layer of metrics. Without this layer, the business user has to go to the technical data team to ask them to help. Usually, it takes a little bit of translation which the business analyst does. For example, the business user says, “I want to run my campaign, and this is what I’d like to see.” The business analyst then can frame that request in terms of data sets, refresh rates, and dashboards.
When the task goes to the data team to pull out the data, put them into a specific format and do some preparation, only then can the business side start consuming the data and get the insights. It can be a very lengthy process.
CDI: Yes, and error-prone.
Yang Li: The result is that the data team today is seen as a bottleneck. The metric store solves this problem by using unified semantics to categorize the data according to the business perspective and enriched in the actual label, so they carry the meaning. In Gartner’s words, it’s a kind of metadata, that is, metadata added on top of the technical data, such that basically, people now know the meaning of data. I think that’s a key difference.
The data innovation can then be realized once the base metrics are well categorized and labeled. For example, if I’m in charge of a supply chain, I may come to the idea that by introducing a new supplier, I can increase the level of competition between suppliers and have the chance to lower the price of a certain material by 5%. And to enable this new vendor, I may have to make some upfront investment, maybe connecting through partners and getting the salesmen aligned. Then to present this whole idea, I need the data to create a business case.
To do that in our metrics store, I can create what we call “derived metrics” on base metrics. Say the base metric is today’s material cost. And I assume there’s 5% less. So I can model it by 95%. I get n an expected lower new material cost. And I can apply some business rules, because the new vendor may only be available in certain areas of the country or in a certain time period. You can apply those business rules plus the upfront investment to enable this. I can determine the ROI and see other analyses. I can do all this in the metrics store without pulling in the technical data team. That’s what I think of as the balance between governance and innovation.
CDI: Thanks for that explanation and the example. You’ve clearly spent a lot of time with businesses and data teams who want to streamline the process of accessing data without compromising on data governance, and all that implies.
Yang Li: Yes, the metrics store solves a lot of problems because in the old days, when the data becomes too big, data teams are busy just storing the data. But you also have to be able to process the data. Then comes analyzing it. Once all these basic steps are done, we can start thinking about the methodology for providing self-service access to it.
CDI: Let’s turn our attention to the business impact of your own product strategy. This Gartner Summit is seen as the best conference to get a feel of where the market’s at. The attendees are trying to figure out where the technology’s at, and the vendors are trying to figure out where the customers are at. What shifts have you observed here? Is there a different business problem or a different way of thinking that customers are talking about?
Yang Li: Definitely. The pandemic, as well as its financial effect, had a negative effect, to be frank. It has led a lot of customers to think about cost. We have been serving in both the US and China. Before, companies didn’t really care about cost. There were a lot of duplicated data constructions. Every organization tries to use data to present its achievement. And overall, they are aligned, of course. But when it comes to my achievement, I like to polish the data in my own way such that my value stands out. When every team is doing that, we get data silos because my way of calculating results is different from yours. And I even don’t want to reuse your data pipeline because I have my own way to interpret. I might not even trust your pipeline because you may change it at any time. I want to secure my outgoing numbers so that they’re stable, accurate, and reliable.
I can give you some concrete numbers on the cost of this duplication of work and data. One of our customers, an online shopping company, started their data as the entry point. Transaction data landed in a landing zone and typically was just a few hundreds of different tables–that’s reasonable for retail. Then because every team has manipulated the data, we see a lot of intermediate tables, aggregated tables in the data lake. All are created and maintained by different teams. The few hundred tables in the landing zone have grown to almost a million tables in the data lake. 100 times inflation. Do we really have so many different ways of analyzing the data? I don’t think so.
Imagine how much work is duplicated, wasted, and how much IT cost and computation power are wasted without governance in place.
CDI: I can indeed imagine that. Is this where the platform’s intelligence comes into play?
Yang Li: The intelligence manifests through auto-acceleration, which is built on sophisticated pattern detection. The platform learns from past actions to suggest future actions, which speeds up the queries. Not only is time reduced, but so is the cost of each query. This query optimization is very complex to engineer–we build a multidimensional model with layers of optimization inside it. For the business user, we hide all that complexity. They can get metrics with one click. The speed is a result of the way we cache the data behind the metrics. The patterns allow us to prioritize and do related precalculations. Later, similar queries are cheaper to run.
CDI: What’s next for Kyligence in terms of your go-to-market strategy of your product roadmap?
Yang Li: Most importantly, we’ll try to sharpen the cost-effectiveness side of the product. And further simplify the product, that is, hide more complexity.
CDI: And looking to your customers, what is the next big challenge that you want to address for your them? What’s the thing that you really want to solve for them?
Yang Li: As I’ve mentioned, I want to help them to be more cost effective. At the same time, we want to create a balance of a governed business-data layer and innovation for them. So I think that’s the challenge because, on the one hand, people are still getting to learn how this works. They’ve just gotten over the stage where data can be stored and processed. They’re still looking for an optimal way to govern the data and to work with it. There have been different attempts. Some have failed. Some are very technical– data warehouse, data lake, ETL, ELT. These are good technologies, but they’re very technical. They haven’t reached the point where the business side is welcoming them and understanding them.
CDI: So to the business side, these technologies are all still plumbing. They just want information and don’t care much about the mechanics of getting it.
Yang Li: That’s the challenge. That’s why we are traveling around the world and talking to different people promoting the benefit of a unified semantic layer or metric store. However you call it, I believe this approach will become very popular.
CDI: Thank you very much for lifting the curtain so that we could see the complexity that you’re shielding business users from. We look forward to talking with you again and learning more about auto-acceleration and the difference it has made for your customers.
Elisabeth Strenger is a Senior Technology Writer at CDInsights.ai.