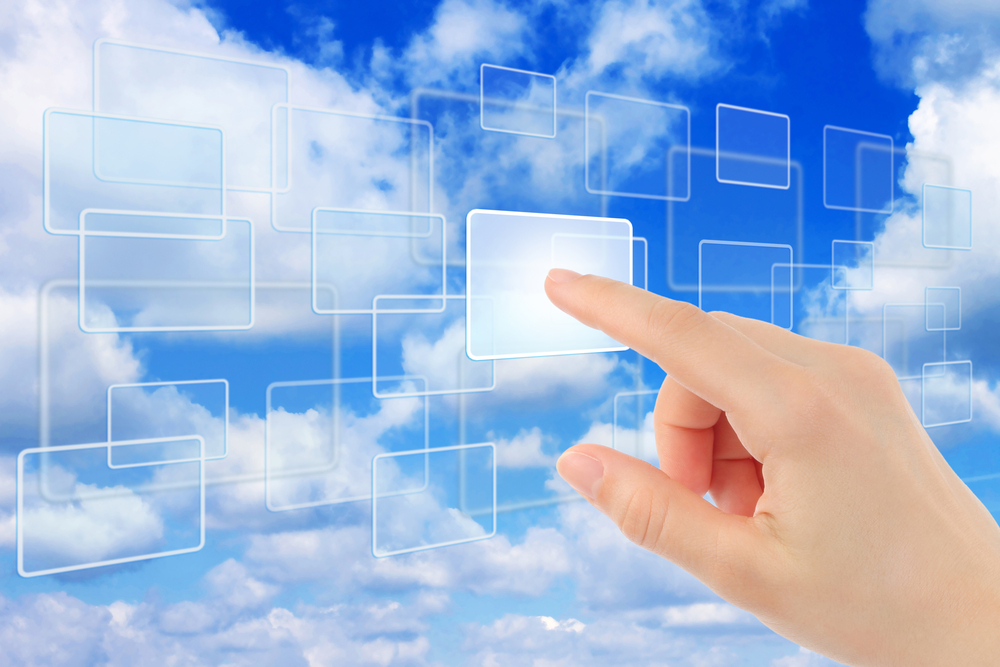
The partner ecosystem of technology vendors and solution providers that has sprung up around Databricks was a prominent part of the recent Databricks Data & AI Summit (DAIS), reflecting the reality that no one uses Databricks in isolation. To solidify its position as foundational data management technology, Databricks has built expansive relationships with those software and hardware providers and consulting organizations that ultimately help customers gain the full value of a data lake.
What distinguishes Databricks’ approach to partnerships from many others is the emphasis on partnering to “complete the workflow” for customers (as summarized by FiveTran’s Michael Bull). This means ttaking a customer-centric view of the partner value chain rather than a technology view which would perhaps focus more on completing a modern data stack checklist. A partnership strategy that wants to make sure that all pieces of a data stack are present and accounted for might not call for the same level of integration, smoothness of use, and variability as partnering for a complete workflow might.
Cloud Data Insights spoke with a number of partners at DAIS to understand how a holistic view of what collection of technology a customer needed to get work done shaped the dynamics of the partner ecosystem. The most direct impact was on the degree of collaboration and the amount of work shared between Databricks and its partners.
Ecosystem Dynamics at Play
Databricks has defined an overarching blueprint for how its partners build out or build on the Databricks modern data stack and complete the entire data lifecycle. They provide data sources, integration, data governance and security, business intelligence, and AI/ML. Others in the ecosystem offer vertical or specialized applications and/or deliver consulting services. “Technology Data Forward” awards recognized partners’ impact in several of these categories.
The Databricks ecosystem seems to orient itself on three main vectors along which partners distribute themselves, emphasizing one or the other or all three::
- Technical alignment
- Complementary market opportunities
- Focus on the customer experience
How Does Technical Alignment Happen?
Certainly not by accident. Nor is it left completely to the dynamics of open-source projects where Databricks and its partners foster open standards that are so necessary if products are to interoperate smoothly.
We believe [that] unless you have open standards it’s always going to be difficult to unify, right? It’s going to have lots of fragmentation. We evaluated a number of these formats…and we see that Parquet is taking off…How many projects have 1 billion downloads per year? …so we’ve been collaborating on the open-source front quite a bit and we need an open format.
See also: Databricks Contributes To Three Open Source Projects
Lindsey Allen, GM of the Databricks Engineering Partnership, Microsoft
Beyond open-source collaboration, Databricks and its partners engage at different levels in the software (or hardware) development process, from sharing product roadmaps to communicating regularly and even at times embedding engineers in each others’ teams. The Databricks Unity Catalog is a good example of the results that can be achieved through this kind of engagement.
The Unity Catalog allows for a federation of sorts of the many data catalog entities that might be touching a customer’s Databricks environment, these catalogs are probably being built with a variety of tools. For partners, the Unity Catalog allows them to unify their own catalogs quite seamlessly. It’s this kind of technology that can be the basis of a powerful data fabric or an effective data mesh strategy for customers. Perhaps the most important benefit that comes out of the Unity Catalog is that security and governance cascade from it to all data products and applications built on the Databricks data.
See also: Cloud Data Management Security Report Shows Progress and 7 Data Lake Best Practices for Effective Data Management
Here are some partners’ perspectives on the importance of the Databricks Unity Catalog to their own offerings and the benefits that are passed on to customers.
The catalog is a really important sort of centralized governance layer that Databricks offers…makes it easy then to surface the metadata. Where did that data come from? Who has access to it? Who’s allowed to see it? Who’s doing what with it? We’ve really focused on that unity catalog integration, which has been fundamental for what any organization is looking for.
That integration facilitates a level of trust.
Michael Bull, Director of Strategic Alliances, FiveTran (Innovation Partner of the Year)
A partner who provides a catalog of catalogs that extends the Unity Catalog’s ability to enable secure federation explains it this way:
[The Unity Catalog is] a governance and a security solution for assets that are used for the data lifecycle and the AI lifecycle. So we catalog the BI metadata, the database metadata, it could be SAP metadata or Salesforce metadata and the Databricks metadata will feed into it. It enables the first pillar of the data-driven culture–self-service analytics, where it’s very simple to find and understand the data.
Diby Malakar, VP Product Management, Alation (Data Governance Partner of the Year)
Sharing Market Opportunities
Fueling many of Databricks’ partnerships are customer use cases that require the smooth operation of several products to address the business challenge at hand. The dynamics flow both ways from partners to Databricks or from Databricks to partners. For example, an enterprise might have standardized on Databricks as their lakehouse platform but now they want to add a security platform or knowledge graph tool to the environment. The solution requires a level of robust integration appropriate to the customer’s data architecture and business requirements. This could be a real-time data pipeline, direct execution in the Databricks environment itself, or a native connector. The ecosystem provides the best-of-breed integration as customers can rely on the Databricks validation of partners’ offerings.
See also: High Performance Data Pipelines for Real-time Decision-making
An area of focus and growth for Databricks is verticals where partners bring together deep experience in solving business challenges with expertise in managing and leveraging data.
One of their partners with a strong focus on retail is Tredence, a solutions and services provider who garnered several awards at the Summit. Tredence and Databricks jointly develop technology to address specific retail use cases such as avoiding out-of-stock scenarios using AI/ML to predict shortages in stores and from suppliers.
The bridge between the business and the platform is getting very, very wide. We bridge the gap and because we know technology and we know exactly the business needs we are able to pivot technology to the right direction…A couple of our solutions are on-boarded as Databricks Brick Builder solutions [e.g. the Revenue Growth Management Framework]. Data science and analytics just providing insights is not enough. It needs to be operationalized, integrated, and built upon for an organization to really benefit and see money out of it.
Lakshmikant LK Gundavarapu, Chief Innovation Officer, Tredence
At the DAIS, Databricks announced the general availability of their Marketplace–an open environment that allows users to use data sets, models, and analytics (i.e., data products). These are sold by an organization or provided at no charge to encourage the use of data collections and models.
To make the data easier to use, notebooks provide context and documentation. The bold aspect of the Marketplace is that there is no requirement to use these data products on Databricks. Users can use the Marketplace on their preferred cloud, data management platform, analytics software, and AI/ML solutions.
Through intensive work on big data and timely renewing external data in the financial services industry, Crux created pipeline solutions that Databricks is using to populate its Marketplace.
The financial services industry [doesn’t] have an exclusive on this problem. It just happens to be where it was most acute first. In enterprise use of AI, AI craves more data to learn and which has external sources. Internal sources weren’t enough [because] there’s too much bias. You need more data–more data for more accuracy, and for more use cases in different verticals.
Jonathan Buckley, CMO, Crux
Sometimes, the difference between industries goes beyond how the business uses data into the de-facto standard technology stack. For manufacturing, most of the world’s manufacturing data flows through SAP applications. One of the launch partners for the Databricks Lakehouse for Manufacturing was Qlik for its experience understanding the SAP data architecture:
If you want to do predictive analytics and machine learning, if you want to bring together SAP with non-SAP data, or if you want to do real-time analytics, you need to get the data from SAP and make it available in a platform like Databricks. That creates a whole slew of complexities such as getting the data out efficiently or delivering it continuously in real-time. Once you bring the SAP data over it is pretty complex.
Itamar Ankorion, SVP of Global Partners and Alliance, Qlik (Data Integration Partner of the Year)
Databricks depends on the expertise of their partners to expand its industry strategy–not just for the technical aspects described by Qlik, but also for their knowledge of specific industries and how they use and shepherd their data through layers of regulations and different expectations around performance, latency, various kinds of analytics and reporting, and user personas–in sum, around the user experience.
Customer Experience
Conversations with over 25 partners at DAIS had a common theme: making things easier for the customer. The first couple of mentions came across as an overused marketing claim. When probing directly for the authenticity of this statement and watching demos and listening to presentations, it became clear that the ecosystem, together with Databricks, could substantiate this claim on both business and technical levels.
From leaving little for a user to grapple with in very tight installation processes for an integrated solution to designing a simplified way to deploy and acquire products and applications, the ecosystem is making strides toward this goal. And it has the broadest definition of “customer” which includes the business owner and end-user, data scientists and data engineers, data product builders and maintainers, governance teams, IT teams, and, refreshingly, the application developer.
The newly launched Lakehouse Apps provides the Databricks infrastructure for developers to use their preferred tools to create and test their applications. Then they can distribute these through the Marketplace and potentially reach 10,000 Databricks customers according to Databricks’ VP of Product Management, Shanku Niyogi. This distribution aspect of the Marketplace addresses many challenges for software developers. It streamlines installation for their end customers and most importantly, provides security and facilitates compliance with local regulations.
Data democratization is on everyone’s lips now. That comes with multiple challenges from scaling infrastructure, refining security and governance policies, increasing data literacy, and emphasizing self-service capabilities, which depend on ease of use.
If a partner’s solution appeals to the broadest possible audience, it increases Databricks’ user count and revenue and gains them mindshare with the end-users. Databricks is in effect deploying its partners as brand ambassadors.
Dataiku explains the dynamics behind the impulse to prioritize ease-of-use:
We want to make all of that underlying technology available to our customers–be it different compute technologies, different storage technologies, different machine learning algorithms, different AI models. And we want to make it all easier to access and easier to use by a greater number of people. So to take Kubernetes, as an example. We also manage Kubernetes clusters for customers where they go in and they just say deploy the data, but they’re interfacing with the underlying cloud service to manage that. So we’re always trying to make it easier for both the architects and the wider population. You end up with 2,000 people [in an organization] who can then work Databricks.
Kurt Muehmel, Strategic Advisor for Everyday AI, Dataiku (AI Partner of the Year)
Databricks has also invested in streamlining the business of software for its partners and the partners’ customers. Through Partner Connect, partners can make acquiring their solutions as frictionless as possible. Here you can try tools and platforms in a live Databricks environment and start evaluating solutions immediately. Integrations available through Partner Connect are validated by Databricks and often have a straightforward getting-started procedure with documentation and other resources that facilitate “test-driving” solutions.
A trusted network of interconnections
An organizational chart of an ecosystem can resemble a hub-and-spoke model where partners engage mostly with the core vendor. Databricks’ ecosystem can best be illustrated as a neural network where layers of interconnections among the partners and Databricks are at play. There were many examples of partners engaging with each other in open-source projects, in user communities, and jointly creating solutions that complete the data workflow or address an industry’s or organization’s challenges. This neural network of partners is not unique, but at DAIS, it was thriving and dynamic, with new technologies, like generative AI, forging more interconnections with existing and new experts.
Elisabeth Strenger is a Senior Technology Writer at CDInsights.ai.