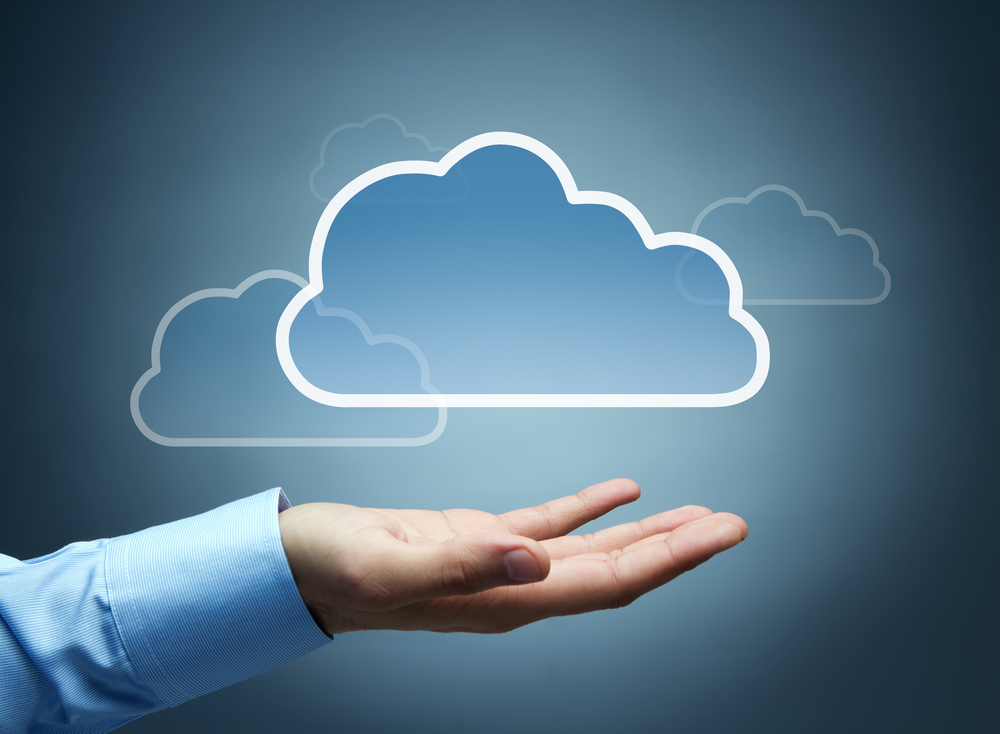
The technology world is full of innovations that take useful aspects of two separate technologies and create a whole new category of products. Clock radios, fax machines, and smartphones stand as popular combinations that changed the lives of many.
“Data lakehouses” have been pitched as one of the newest examples of this type of innovation. Backers describe it as a cross between a big, hard-to-access data lake and a costly, limited-functionality data warehouse. They say that data lakehouses combine the best features of data lakes and data warehouses: the flexibility and relatively low cost of a data lake, coupled with the ease of access and support for enterprise analytics capabilities found in data warehouses.
It’s a reasonable argument based on the needs in the marketplace and the shortcomings displayed in the age of unstructured (or semi-structured) data. But are data lakehouses really poised to become the market drivers proponents say they will? Or are they just another passing fad that’s making noise today but will be replaced by a new, more targeted innovation tomorrow?
The answer will impact the strategies of large numbers of enterprises looking for solutions to manage data in a variety of formats, including those that could potentially be analyzed by artificial intelligence (AI) and machine learning (ML) tools, such as text, images, video, and audio.
See also: What is a Data Lakehouse?
It’s a bird! It’s a plane! It’s …
Today’s rapidly expanding data landscape is being served not only by data lakes and data warehouses but also by data hubs and analytics hubs (with the functionality of these two platforms as generally nonexistent in data warehouses or lakes). What are all of these mechanisms? And how do they relate to each other?
Let’s start with a data lake. A data lake is the upstream location where all of the organization’s data flows. Data lives there in its raw state – either unstructured or structured, in image files, PDFs, databases, and other formats. Data lakes can typically ingest and manage almost any type of data, and as exemplified by Hadoop (historically the most popular type of data lake) and, more recently, object stores like S3, ADLS, and Google Cloud Store, they provide tools for enriching, querying, and analyzing the data they hold.
Data lakes have historically been used to explore new ways of mining, combining, and analyzing data that was thrown out or not used as part of day-to-day business processes. In other words, it was applied either to operational data that is no longer in service or to data that may be considered in the future for operational use but is nonetheless currently in exploratory mode.
See also: Okay, Your Data Is in The Cloud. Now What?
A data warehouse tends to support long-standing datasets that represent fundamental, core data that runs the business: customer records, supply chain bills of materials, and so forth. Most of this data is highly structured but increasingly has semi-structured elements, incrementally built over time from multiple downstream data source silos. Changes to how the data is used can be time-consuming – not because of the data itself but because of the intricacies of how, where, and by whom it’s being used. New datasets – possibly after exploratory phases of work in the data lake – are made available for more regular, and routine analytics in the data warehouse, provided it can accommodate the size and structure of that data.
Data warehouses are increasingly incorporating data streams and advanced analytics on both historical batch and real-time data streams. In general, data warehouses also differ from data lakes in that they require some sort of data hub technology to prepare the data for ingestion.
But how do hubs come into play? A data hub is a gateway through which virtual or physical data can be merged, transformed, and enriched for passage to another destination. That destination might be an application or a database or some other kind of repository (such as a data lake or data warehouse) either for use by applications as a part of their ongoing business/operational process or by an analytics platform as a feedback loop on the process – automated or human decision support, exception handling, etc.
Read the rest of this article on RTInsights.
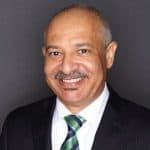