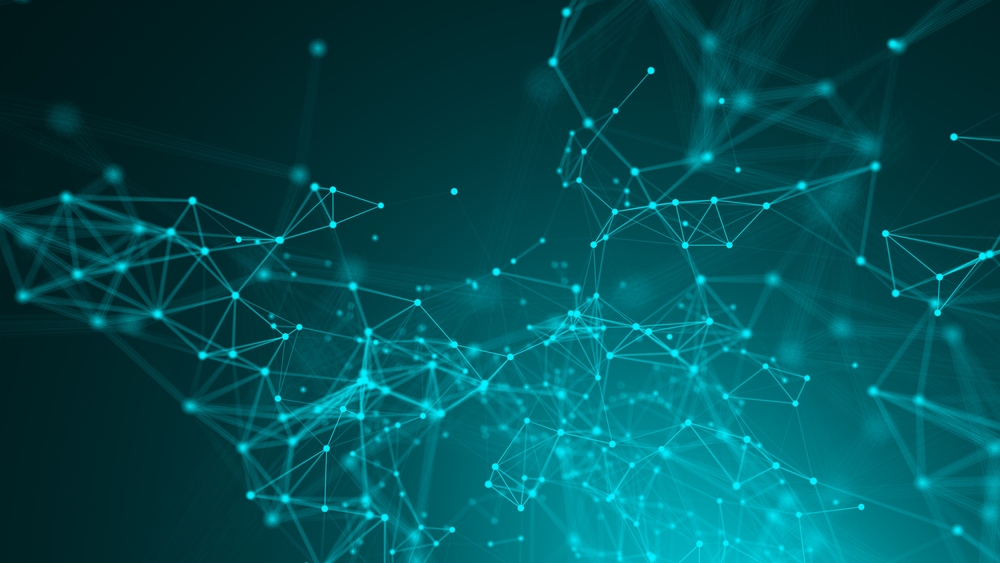
Data mesh is once again on everyone’s mind thanks to press from industry analysts like Gartner and McKinsey. It promises to help companies finally become data-oriented if they can only figure out how to implement and execute it within their own data structures. In a fascinating webinar presented by Data Science Salon, “Data Mesh: From Concept to Reality,” speakers Matheus Espanhol of BairesDev, Jason Pohl of Databricks, and Jon So of Monte Carlo demonstrate just how possible it is to leverage this decentralized data architecture approach using Databricks and Monte Carlo tools.
The four principles of a data mesh
In order to make the most of this concept, you must understand the four principles of a data mesh.
- Data domain ownership: Companies must host and serve data in an easily consumable way
- Data as a product: Application of product-making to data, i.e., easily discoverable and read, as well as versioning and security policies.
- Self-serve data: Tools and user-friendly interfaces.
- Federated governance: An overarching set of policies to govern operations
These are the foundation of a well-executed data mesh. Companies must have this foundation in place before they can build a functioning data mesh.
Technical challenges of managing data
The companies participating in this webinar understood the challenges of becoming data-driven. They experienced challenges in scale, as well as the limits of their existing infrastructures. In addition, a lack of trust and quality prevented real data-driven decision-making.
For Bairesdev, executing a data mesh required planning and restructuring their existing technology. And it wasn’t easy. The company includes over 5,000 engineers across 36 countries and delivers its services to a host of brands around the globe. Their solution needed to cause as little disruption as possible while improving the insights given to them by big data so they could help their customers in turn.
The team evaluated solutions to build a custom data mesh
BairesDev looked at some of its most perplexing challenges and noticed an overlap with the four foundational requirements of a data mesh. This helped make decisions a little easier because the team knew and understood what they were working towards.
- The company had strong autonomy and domain ownership already in place. It was able to find and define data owners.
- However, consumers didn’t trust the data. A lack of observability and no data product role kept performance and availability to a minimum.
- The company had good practices in privacy but centralized metadata management and global policies.
Implementation presented its own challenges, but planning and creativity helped
The goal was to reduce complexity to begin the journey towards a data mesh. The company purposefully chose managed options to implement automation. This would help reduce the time to market for data products. Tools such as Fivetran, Monte Carlo, and Databricks provided these capabilities.
The company also needed to reduce the complexity and scope. Kafka Connector Manager and Databricks CD provided automated integration tools and supported the creation of new architecture without building from scratch.
- Databricks: The lakehouse construction simplified the architecture and helped cover distinct domain needs.
- Monte Carlo: Incident IQ helps with root cause analysis and encourages data discoverability. Users were able to maintain high-quality data products, including shared options.
The two keys of success: Data lakehouses and data observability
The lakehouse is simple, multi-cloud, and open. The lakehouse is a complementary, not competing, technology. In addition, the Databricks Unity Catalog allows the administration to manage and authenticate users from a central location.
Another tool for executing a data mesh is Delta Sharing, the first open protocol for data sharing. Users can share data within their existing data lake with partners, suppliers, or even customers outside the identity provider. It allows users to scale their data mesh and integrate with other users and tools.
As for data observability, Monte Carlo integrates with the Databricks Lakehouse. It automatically notifies domain or data team owners of anomalies and nudges teams to resolve the incident. Monte Carlo tools also help them understand how changes downstream or in the schema will affect the overall system.
It can automate observability markers and facilitate the self-serve portion of a data mesh. These are preprogrammed to check for common issues and work out of the box. These are customizable through the platform and ensures that even a decentralized architecture offers a cohesive governance strategy.
Two common ways to organize a data mesh using Databricks
Companies must decide to balance autonomy with complexity.
Harmonized
This is the truest form of a data mesh. It requires each domain to have the skills to manage the end-to-end data lifecycle but can create inefficiencies if there is a high level of data reuse.
Hub-and-Spoke
This option offers a hybrid data mesh with some centralization. If there are a large number of domains, it can reduce data sharing and management overheads. However, it blurs the boundaries around a truly decentralized system.
Data Mesh is attainable and actionable
The webinar clarifies how companies can implement new concepts, such as the data mesh, to transform how they handle data. It isn’t just a conceptual architecture but one that companies can achieve with planning and the right tools.
To view the entire webinar on demand and see more details about how the pieces fit together, visit the Data Science Salon.
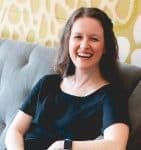
Elizabeth Wallace is a Nashville-based freelance writer with a soft spot for data science and AI and a background in linguistics. She spent 13 years teaching language in higher ed and now helps startups and other organizations explain – clearly – what it is they do.