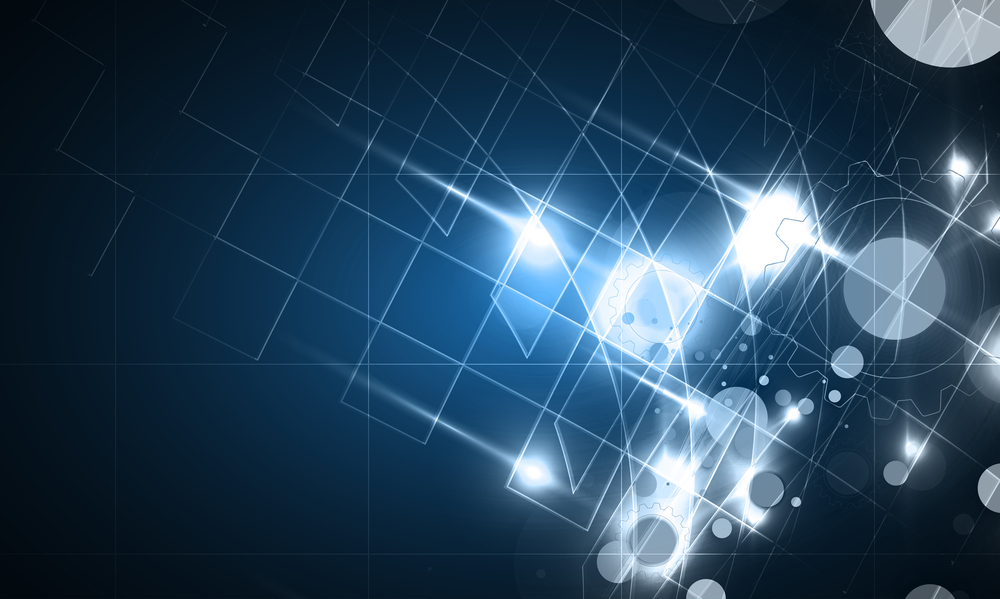
This article is sponsored and originally appeared on Ascend.io.
Ready or not, data mesh is fast becoming an indispensable part of the data landscape. As data leaders, the question isn’t if you’ll cross paths with this emerging architectural pattern. The question is when.
A shift this monumental can seem daunting, often leading to analysis paralysis, overthinking, or other implementation delays. This is where we want to step in. While the journey will differ from company to company — because of their unique business and data needs — there are fundamental principles that provide a blueprint for action.
In this article, we provide practical, actionable steps to kick-start your data mesh implementation using the well-established approach of managing People, Processes, and Technology. This isn’t another theoretical deep dive. Consider this your primer to stop overthinking, start acting, and truly harness the power of data mesh.
Establishing the Baseline for Data Mesh Implementation
Data teams everywhere are leaning in, eager to figure out how data mesh can help. Sure, we’ve all seen trends come and go, causing a bit of chaos as we shift strategies and adjust our tech stacks. But something about data mesh feels different, doesn’t it?
For one, data mesh tackles the real headaches caused by an overburdened data lake and the annoying game of tag that’s too often played between the people who make data, the ones who use it, and everyone else caught in the middle. It feels like data mesh showed up just in time, as we’re all hustling to refine our data platform strategies to build better datasets, dashboards, analytical apps, algorithms, or, in short, data products.
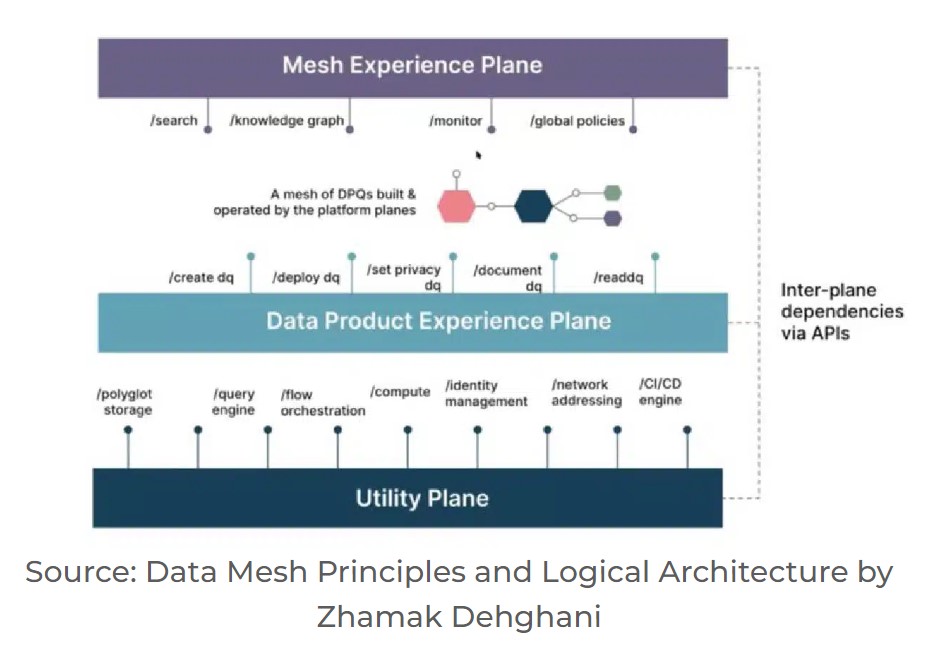
In this article, we won’t be diving into the deep end of what data mesh is. We’ve got plenty of resources for that — check out the Data Mesh Learning community or our previous articles:
- What is a Data Mesh? — And Why You Might Consider Building One
- Benefits of Data Mesh and Top Examples to Unlock Success
- Data Mesh vs. Data Fabric: Which One Is Right for You?
The tricky part isn’t understanding what data mesh is — it’s figuring out how to put it to work for your organization. So, the million-dollar question is, where do we begin this journey?
While the data mesh concept is relatively new, we can leverage the established framework of managing People, Processes, and Technology to successfully guide the implementation. This framework has long been a trusted approach to complex transformations. Each of its three pillars offers a specific focus area that is essential for the successful implementation of any large-scale change, including a data mesh.
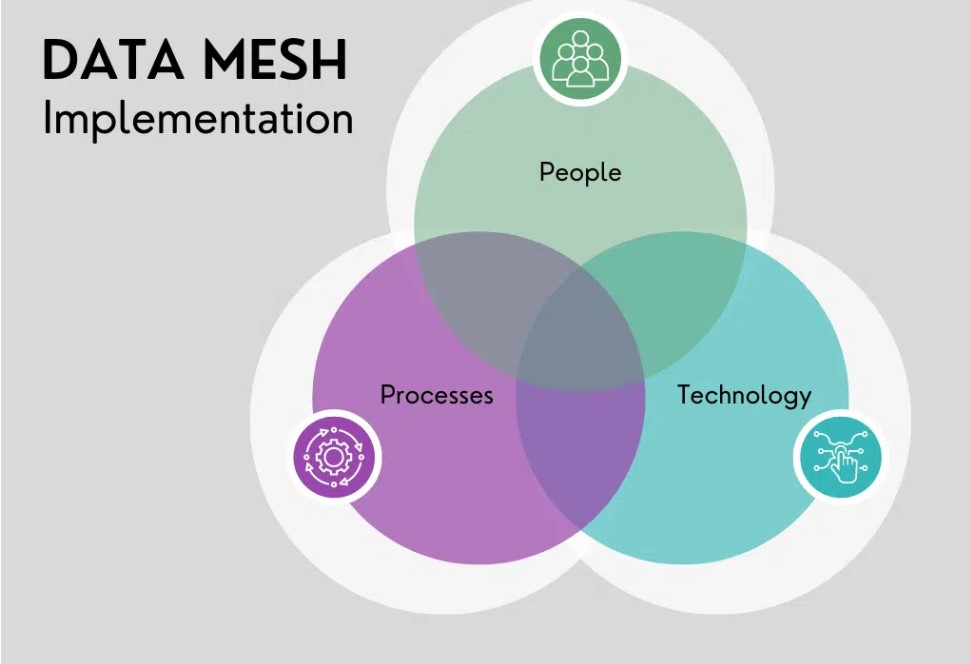
People: Defining your Domains, Assessing Domain Maturity, and Selecting Your First Partner(s)
The data mesh model inherently pushes for domain-oriented decentralization, which implies a significant focus on the people within those domains. Establishing ownership and understanding the varying technical maturity levels across these domains is the initial, crucial step.
Identifying Domains
The shift towards a data mesh implies treating each domain as its own miniature data ecosystem. Defining this organizational architecture should be first.
Here are a few of the most common issues with data meshes:
- Identify data domains within your organization. These could range from functional groups like sales, marketing, and finance, to broader LOB units which would likely be further broken down into sub-domains. Your first pass may not be perfect but will be the start of the organizational architecture. Understand the role each domain plays in creating, maintaining, and consuming data products.
- Find a partner within each domain – someone influential who can champion the cause of the data mesh within their domain, making the transition smoother.
Assessing Domain Maturity
One major overlooked thread when it comes to implementing a mesh is to have a well-defined maturity model. By using such a model you can create repeatable patterns and process to support and move Domains up the maturity scale. At the end of the day, the best data mesh implementation pulls the entire organization in to participate, not just those that are have the dollars and skills to do so.
- Use the same people, process, and technology to create a maturity model. A good starting point looks at the following dimensions:
- People: What level of technical and curation skill does the domain have?
- Process: Are there current processes in place that already support the move to Data Product creation and curation
- Technology: What level of platforms and tools exist?
- Rank and categorize domains that you may already know based on the maturity model.
Select Your First Partners
This is the key to moving quickly to show the value in continued investment in a mesh. Using your domain assessment combined with the relationships you already have, choose two partners that are well up the maturity scale to begin this journey with you. As part of this, it’s key to create a Steering Committee.
Process: Building Robust Systems from Data Governance to Product Publishing
Just as a well-oiled machine needs precise processes, a successful data mesh needs to establish and adhere to processes regarding data governance, data curation, and data product publishing.
Implementing Data Governance
Effective data governance is the backbone of any data management strategy, and even more so for a decentralized approach like data mesh. A robust data governance framework is crucial to ensure data quality, manage data access, and maintain compliance with regulatory requirements.
Establish clear data governance policies.
The policies should outline rules and standards for data. These should be explicit and prescriptive, addressing the 5 aspects below:
- Domain and business key definitions: Clearly define your business keys and the domains they belong to. It ensures everyone in the organization speaks the same data language.
- Security access and roles: Define who has access to what data and the extent of their permissions. It is essential for maintaining the integrity and confidentiality of your data.
- Data quality expectations and minimum acceptable criteria: Set standards for what constitutes acceptable data quality. This helps maintain the reliability of data products across domains.
- Contractual data obligations and acceptable use standards: This includes rules for sharing, aggregating, or other usage of data to avoid legal complications.
- Data product sharing and version management: Establish a policy for managing the lifecycle of data products.
Promote cross-domain collaboration.
Encourage different domains to collaborate on establishing and enforcing data governance policies. This collaboration ensures consistency and compliance across the data mesh implementation.
Dealing with regulatory compliance can be challenging for individual domains. This is why core IT often still handles a significant part of data management. Therefore, consider providing specific guidance or resources to help domains understand and navigate compliance implications.
Don’t forget to publish business key definitions for relating domain data and maintain a simple-to-use catalog of domain data. These practices can support data users across domains in efficiently locating and utilizing the data they need.
Streamlining Data Curation
Data curation is the process of organizing and defining data in a way that maintains its usefulness over time. It’s crucial to have a consistent approach to data curation across all domains.
Establish standardized processes and guidelines for curating data products.
To ensure consistency in the data product definitions across domains, these guidelines should at least cover:
- Metadata standards: Define a standard set of metadata to accompany every data product. This might include information about the data source, the type of data, the date of creation, and any relevant context or description.
- Data validation checks: Outline a systematic approach to ensuring data accuracy, completeness, and consistency. This might involve data checks at different stages of the data lifecycle.
- Data lineage documentation: Establish a clear process for tracking the journey of data from its origin to its current state. This is vital for understanding the history and reliability of your data products.
- Data documentation best practices: Define a standard for documenting data products. This should include how to describe data products, how to document any transformations or modifications made to the data, and any other relevant notes or comments.
Train domain teams in these processes.
Providing detailed training ensures the guidelines and processes are effectively followed. Make sure that training materials are accessible and easy to understand and consider offering regular refresher courses to account for any updates or changes to the processes.
Navigating Data Product Publishing
Having a clear process for data product publishing is vital to avoid any hiccups in the mesh due to changing data product definitions.
Develop a data product lifecycle framework.
This framework should guide the entire lifecycle of data products, from creation to retirement. Key elements to consider when working on the data mesh implementation include:
- Creation: Define the processes involved in the creation of new data products, such as data collection, validation, and initial documentation.
- Versioning: Establish a versioning system to track different iterations of a data product, enabling users to understand the evolution of the product and helping prevent confusion or misuse of outdated versions.
- Updating: Set out clear procedures for making updates to existing data products. This should include steps for documenting changes, notifying users, and ensuring the updated product meets established quality standards.
- Retirement: Outline the steps to decommission a data product, which could involve archiving the data, notifying users, and updating any related documentation or metadata.
Implement a publishing approval process.
Create a process to ensure new data products or updates meet quality standards before being published. Key elements include:
- Quality checks: Set up systematic checks to validate the quality of the data product based on your established criteria.
- Approval workflow: Define who is responsible for reviewing and approving new data products or updates. This might involve multiple levels of review depending on the complexity and impact of the data product.
- Notification system: Establish a system to notify relevant stakeholders once a data product is approved and published. This could be through email notifications, updates on your data catalog, or automated alerts through your data platform.
If you need further guidance on treating data as a product in the context of data mesh, we recommend reading our article Essential Capabilities to Treat Data as a Product.
Technology: Laying the Foundation with Storage, Build Plane, and Sharing Layer
The technological infrastructure forms the backbone of a data mesh. It is crucial to have a reliable storage and compute layer, a unified build plane, and a real, user-friendly sharing layer.
Building a Resilient Storage and Compute Layer
The storage and compute layer is where data is housed and processed, forming the base of your data mesh.
- Decide on a centralized or decentralized approach. Consider whether a centralized storage and compute layer would work best for your organization, or whether allowing each domain to use their existing infrastructure would be more practical.
- Evaluate your infrastructure. Understand your organization’s technical capabilities and existing infrastructure when deciding on the storage and compute layer.
Standardizing the Build Plane
The build plane is where the data products are built, refined, and maintained. It’s important to have a consistent and user-friendly build plane for a successful data mesh implementation.
- Aim to consolidate your build plane onto a single platform. A unified platform brings the benefit of a shared, familiar environment for data product development across different domains.
- Having a single platform is particularly advantageous as it provides a consistent user experience, helping users from various domains navigate and use the tools efficiently and effectively.
The choice of platform should accommodate users of varying technical capabilities. Ensuring that non-technical users can operate effectively within the platform broadens the range of users who can contribute to and leverage the benefits of the data mesh.
Streamlining the Sharing Layer
The sharing layer is the bridge that connects users to data products in the data mesh. It not only facilitates the discovery, connection, and use of data products but also ensures that the diligent work put into creating data products reaches its intended audience. As such, it’s one of the most challenging aspects of the data mesh, primarily due to the need for dynamic data sharing and change management, particularly as data products evolve.
Dynamic data sharing:
One of the greatest challenges is establishing a mechanism for dynamic data sharing. This process involves creating a system that automatically updates data for everyone who has subscribed whenever there are changes in the data products. This requires a tight integration between the sharing layer and the data product versioning system to ensure subscribers always have access to the most updated data.
Accessible and user-friendly design:
It is essential to ensure the sharing layer is intuitive and easy to navigate. Users should be able to find and connect to data products with ease, making the discovery and connection process as frictionless as possible.
Prioritizing discoverability:
The effectiveness of a data mesh greatly relies on the ease with which users can find and utilize existing data products. Therefore, investing in features that enhance discoverability, such as advanced search functions or a well-structured data catalog, is critical.
Integration with data governance:
Lastly, the technical sharing capability should be closely aligned with data governance processes. This helps simplify change management when data products are updated, ensuring that changes are reflected promptly and accurately across all instances where the data product is used. Such integration also ensures that any changes comply with established data governance policies, maintaining the quality and reliability of your data products.
Taking the First Steps Towards Data Mesh Implementation
With countless resources at our disposal, the theory surrounding data mesh is well-established and readily available. However, simply understanding the principles of data mesh isn’t enough to spark transformative change. Real progress comes when we move beyond comprehension to the realm of application.
This article has laid out the foundational principles integral to embarking on a data mesh journey. By treating it as a comprehensive project plan and systematically addressing the aspects of people, processes, and technology, the task becomes much more manageable.
While the data mesh is a relatively new concept, the framework we’ve discussed is a classic approach to getting things done and can be effectively applied to implement a data mesh. As you navigate this journey, remember that the ultimate goal of a data mesh is to unlock the true potential of your data, enabling rapid insights and more effective decision-making across your organization.
Jon Osborn is Field CTO at Ascend.io.