Companies today are facing an overwhelming challenge in dealing with large, complex data sets that are being generated at increasingly faster rates. In many cases, they are turning to DataOps for help.
Why? With a growing demand for speed and agility, as well as the influx of new and disruptive technologies like the Internet of Things (IoT), cloud computing, and the power of Big Data integrated into everyday use, companies are generating at least 50 times more data than they were just five years ago.
Additionally, the pandemic has spurred many organizations to become fully digital – adding the complexity of data access, use, and storage across the network with analytics on use, security, and other insight generation into the mix. According to a recent survey, more than 80 percent of enterprises have a hybrid cloud or multi-cloud strategy, further exacerbating this complexity for IT teams. With more data comes a need for greater efficiency – and data experts are in high demand.
Organizations that want to win big must act and adapt quickly to deploy and scale new software and solutions that provide customers with a superior experience and satisfy their rapidly evolving needs. To do that, they also must be able to rapidly aggregate, integrate and analyze data sources – something they’ve long struggled to do, even when data was centralized in on-premises data centers. Now, data is distributed across multiple clouds and out to the edge with IoT, mobile and sensor devices. To further complicate matters, this data often needs to be used by large, dispersed workforces, which means data must be able to be delivered quickly and securely.
To manage this increasing complexity, many organizations are adopting DataOps teams, leveraging the same agile methodology as DevOps, which has transformed the speed and capabilities of software development teams over the last decade. Organizations continuously aggregate, transform, enrich, and deliver reliable data, often via automated processes, so that the business can make faster, data-driven decisions. DataOps is critical to addressing the challenges involved in acquiring, storing, and governing data; it also provides enterprises with cost-effective options to securely manage increasingly large dispersed, dynamic and varied datasets.
DataOps for optimizing the use and value of data pipelines
The concept of DevOps has been around for a little over a decade, but DataOps has been around for less than three years (not counting Manifestos as origin dates, particularly for DevOps). In the early days of DevOps, it was thought of as a radical new methodology with resistance from IT and Developers for totally separate reasons. Developers were resistant to the scrutiny and teamwork required by small incremental, transparent changes that rapidly went through test to deploy, and IT was resistant to the rapid speed of change DevOps represented. All of this apprehension is in the past, and DevOps has now caught on with IT, development, quality assurance, and product management teams. In combination with agile and lean methodologies, the technologies, processes, and cultural changes have taken hold and only strengthened over the past two years through COVID-19.
Yet, with all the success of DevOps, the ability to rapidly develop, deploy and iterate on applications and their underlying IT environment, it doesn’t fully address the issues with the data associated with those applications or the data necessary to know if those applications are optimally delivering on the business objectives. To make matters more pressing, the underlying environments of multi-cloud environments with SaaS applications and data lakes, leveraging Kubernetes and VM deployment of microservices architectures have only meant that in addition to long-standing issues around siloed departmental data, inflexible legacy data warehouses and database and document repository sprawl, data pipelines associated with applications are now far more complex and distributed across multiple edge, on-premises, and cloud platforms.
Just like DevOps, DataOps isn’t a product but rather a cultural shift supported by many existing products. Just as DevOps focuses on the Application Lifecycle from development through testing to deployment with the application as the focal point, DataOps focuses on the data pipeline from data ingestion into the pipeline (generally in some hub), through enrichment (either in the application or in a data management platform), through use in an application or analytics platform where the data is the focal point. The two are tied together in that the technologies used by the developers for collaboration, storing, and managing code and its development, testing, and release for deployment are largely the same.
It’s the orchestration and data analytics additions to this set of technology that DataOps brings to the table, with a focus on different members of the IT and Line of Business teams, specifically the Data Integration Specialists and Database Administrators, Data Engineers, Data Scientists, and Business Analysts, tasked with continuous improvements to the value the businesses are getting from their data. While Developers and ITOps are part of the team and applications are part of the focus, the real attention is to the data and those most involved in building the data model, cataloging the data, and analytics assets generated with the data pipeline. Like DevOps, the goals are agility, transparency, resiliency, collaboration, and continuous improvements.
Rethinking your data management
Most enterprises have invested in significant data infrastructure to extract, load, and store their data in tools such as data warehouses, data lakes based on Hadoop, and data marts. These data management and analytics platforms have been utilized to model data but have been typically implemented with a predefined and often rigid data model on partial sets of data. For example, enterprise data warehouses tend to be used for long-term storage and periodic operational analysis of historical data. Data lakes tend to be used in the research and development of new ways to look at new data, such as applying AI to social media or clickstreams of data. In both cases, the data in these platforms are not readily accessible by anyone outside of the IT organization. The same lack of self-service applies to the tools that are used to ingest data into these systems as well.
DevOps and DataOps are two different things, but what they have in common is that the tools had to change to facilitate real change to the process and culture. The same will be needed for DataOps – and like DevOps, we should expect that it will not be a single domino effect or straight line.
Think about the distinction we made above in stating that the enterprise data warehouse is involved with operational data and the data lake is involved with exploratory data. These are often seen as two separate data pipelines with separate tools, both often disintermediating the business because they don’t have self-service access. But the other issue is there is often not a consolidated data model that is understood and accessible for data sources nor a common catalog of data assets or analytic assets that are generated on the other side of the pipeline. In the future, for the DataOps process to truly make a difference for enterprises, this must change. Enterprise data warehouses and data lakes must be more accessible and tied to a common set of integration tools, a common enterprise-wide data model with catalogs of all assets and accessible by the cross-functional IT and business team.
In summary, DevOps may have spurred DataOps, but they are not one and the same. While there is overlap for IT and Development teams, the focus for DataOps is an entirely separate set of technology, and the data management platform components target a separate set of virtual team members for an entirely different mission: getting more value from the data for the business. There is a closed feedback loop to the applications, ensuring they leverage the data better to improve efficiencies and outcomes, but it’s centered on the data, not the applications and their infrastructure.
The technological revolution is creating unprecedented opportunities to move forward leveraging data. By analyzing and acting on the data that powers modern business, there is value in every dimension of our organizations. As is the case with every transformation, the benefits of building an integrated, self-service analytics environment will be realized by “users” rather than IT. For the data science and analytics staff to be successful, they must be exposed to all the necessary technology and processes to support the effective use of data. Leveraging the power of data science, business leaders can achieve operational excellence and compete effectively against the growing wave of competitors. DataOps creates a culture and process with the right technology to make that happen.
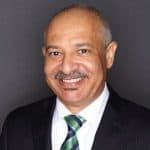