Companies increasingly need to perform analytics on all forms of data, including unstructured, structured, historical, real-time, or any combination of the above. The data volumes frequently are enormous, and insights must be derived promptly to take quick actions. There are many technical challenges, performance problems, and data privacy concerns. RTInsights recently sat down with Joy King, VP of Vertica Product & GTM Strategy, to sort through the issues companies face and how a unified analytics warehouse can help. Here is a summary of our conversation:
RTInsights: What is a unified analytics warehouse?
King: The most important thing about a unified analytics warehouse is what it’s not. And that, as many people notice, is that “unified analytics warehouse” doesn’t have the word data in it. Now there’s a reason for that. The key here is to unify the analytics and not focus on all the data in a single location. Because frankly, that’s not viable anymore.
Organizations have the need for very resilient, very reliable, high-performance analytics data warehouses and the need for data repositories, whether you call them data lakes, data swamps, or whatever you like to call it. The reality is that in many cases, data lakes are a source of complex data types, open-source data formats, and other applications that need those data. We need data in those formats. And many of our data science projects use data in complex data types with languages like Python and Jupyter notebooks.
The key is to unify the analytics, let the data scientist or the business analyst use the tool or language they might need to analyze data without requiring all the data to be in one place. That is why a unified analytics warehouse is missing a word that most people assume, and that’s data.
RTInsights: What is driving the need for it now?
King: The factor driving the need for a unified analytics warehouse has been an issue for years. Let’s think about this. First, we had the first-generation appliance back in the 1980s. Plug it in, put all your data there. We’ll take it from there. That became a complicated and expensive architecture. So, what I call the poor innocent elephant entered the world: HDFS [Hadoop Distributed File System]. She was designed to be a highly distributed file store. That’s what Hadoop was. But as I often say, capitalism intervened, and Hadoop was asked to become an entire zoo. The poor elephant was wonderfully functional, but she was asked to become a database, a SQL query engine, a data science lab, a transactional system, and this and that. And guess what? That didn’t work. At the same time, the public clouds entered the picture, and cloud object storage became another set of data repositories.
So, what you had was a poor elephant that couldn’t deliver on the promises of a total zoo combined with the cloud object storage. Now more than ever, you have silos of data, but a massive need to get to, not just real-time analytics, but predictive and proactive analytics. It’s wonderful to talk about advanced analytics, but if you’re only doing that on a subset of data, what are the chances that you’re going to be accurate?
Alternatively, if you’re doing the analytics on a massive amount of data, but without the performance of something like a massively parallel processing architecture, if you’re highly accurate, two weeks late, that’s not helpful either. Today we’re all focusing on predictive and proactive analytics. You need the full scale of data, the full performance, and the ability to unify the analytics across data repositories without thinking that somehow you can forklift petabytes of data overnight into a different location. Now more than ever, because of the volume of data, the performance required, and the different formats of data, unified analytics is the key to reaching the predictive and proactive outcome.
What I mean by proactive is that if my predictions tell me that there’s an outcome that I need to influence, I want to take proactive action to positively influence that outcome. Proactive means that if this customer has a high risk of churn, or if this looks like the potential for fraud, I want to have the opportunity to take an automated action in time to prevent that.
In a way, it’s prescriptive analytics with the time element. It is already what many, many of the industry disruptors are doing. They must do that. If you think about just from a security point of view and a security center, you can have cybersecurity experts staring at screens, getting notifications. But if you consider the volume level, some of that must be automated, built into the analytics process. It can’t wait for somebody to respond to that red flag. That’s proactive – built into the analytics process and powered by machine learning. That’s the way I think of it.
RTInsights: What is the difference between a unified analytics warehouse and a data lake house?
King: That is a little bit like asking “What’s the difference between Hadoop, originally, and the zoo?” There are two sides to this race to a unified analytics warehouse, and there’s an excellent white paper out by the analyst firm EMA. In the race to a unified analytics warehouse, both sides of the aisle see the need for unified analytics. It’s not that the data warehouses or database management companies don’t get it or that the Databricks or the data lake side doesn’t get it. They all do.
How do you get there? Well, the first question is how easy is it to take a data lake, which was built for highly distributed low-cost storage, and make it a resilient, high-performance, secure database? We all know that that’s a bit of a journey.
Now on the other side, the database side, similarly, it’s not easy. You’ve built your world around a proprietary data format, and suddenly you are opening the gates. Take some of the cloud-owned contemporary players. They are opening the gate very wide, but what are they opening it for? They’re opening it for data loading, making it as easy as possible to put all the data, where? In one place. Put it here in our format, and we’ll take it from there.
What we do is open the doors the other way. Vertica reads ORC or Parquet directly in external data lakes using communal storage options like S3 or HDFS without moving the data. So, the difference between a data lake and a unified analytics warehouse is that the unified analytics warehouse has all the advantages of an ANSI SQL data warehouse database. It is very secure, resilient, and has reliable performance. And it unifies that with the advantages of data lakes with open-source formats, including complex data types. It makes sense to keep the data in those data lakes, but it still needs to be joined with other data. And by joined, I can mean either the English word joined or the SQL JOIN function to provide a single unified analytics outcome.
And I would mention one other key thing here. One of the most important things for all of us in the world of machine learning is the ability to replicate a model outcome. It can be very dangerous not to. There was a very famous story. You may recall when Steve Wozniak, one of Apple’s founders, and his wife both applied for credit. His wife was given a significantly lower credit limit, despite reporting the same income and having a joint bank account.
There was some concern that gender might have played a role. What did the bank need to do to protect itself? It needed to be able to replicate the model to show how the decision was made. But what happened? They couldn’t replicate the model, so they couldn’t prove it was not gender, or it was. That is one of the most important elements of a unified analytics warehouse, along with the scale, using all the data, and the model’s security to prove the outcome after it has had an impact. Because frankly, the PR cost alone for that one story outweighed every other advantage that bank might’ve gotten from whatever technology it was using.
RTInsights: To use a unified analytics warehouse, would all data and analytics need to be on a cloud?
King: The answer to that is obviously, no. A unified analytics warehouse must not, not should not, must not be constrained by the underlying infrastructure. The public clouds are a critical component of our IT world. Not just one cloud but multiple clouds. And we know whether it’s based on privacy, security, or regulations in that country, that some use cases will remain on-premises. That means most organizations will need to plan for a hybrid model. And maintaining the flexibility to change your deployment model is just smart planning.
The underlying infrastructure must not interfere with a unified analytics outcome. And frankly, as any good negotiator knows, it’s very dangerous to put all your eggs in one basket and expect to have any negotiating power going forward with the people who own the basket. So, the answer is absolutely not, for a lot of reasons, but the most important reason is that you must not be reliant on a single underlying infrastructure.
RTInsights: Is a unified analytics warehouse just for companies that have “big data,” hundreds of terabytes of data?
King: The answer is that ultimately, a unified analytics warehouse, as we talked about before, is about unifying analytics and keeping data where it makes the most sense, often having some data in open-source formats and complex data types in data lakes, and having more high-performance, near real-time data in a data warehouse. Neither of those is tied to size. But here’s what I would tell you: most successful companies today, even if they start small, will scale big. I’ll give you two examples. There was this company back in 2007. Nobody knew how to say its name. Was it Yubur or Uber? They purchased five terabytes of Vertica. Well, let’s just say they’re a little bit bigger than that now.
In addition, we have a customer that you may or may not have heard of called Climate Corp. Climate Corp. was an entrepreneurial company, and personally, something I’m very proud of, a company that focused on Ag-Tech. The field is about technology for agriculture and focuses on optimizing farming using sensor data from farm equipment, historical data based on production, and success in different farms, combined with weather data to help farmers be more efficient.
It turns out that the amount of farmland we have on the planet is shrinking, and the amount of people we need to feed is growing. So, it would be nice if we optimized the use of the available land. Climate Corp. became a leader in that field. It started small. Then, I guarantee you’ve heard of the company that I’m going to mention next. Bayer acquired Climate Corp. through its acquisition of Monsanto. Now Climate Corp. is big.
So, it is absolutely true that Vertica brings great value to the unified analytics warehouse not just by unifying the analytics, but also with performance at high scale, dealing with data sizes even beyond terabytes to petabytes. But we must remember that most data-driven companies that scale that big start out small. And even if they don’t scale up that big, having small amounts of data in a data lake, for reasons that data lakes do make sense, and small amounts of data in a data warehouse format, and unifying the analytics is equally important for a small use case as it is for a large one.
RTInsights: What are some examples of companies that use a unified analytics warehouse, and how do they use it?
King: There are many, many companies. I just mentioned two. There are others you might find interesting. There is a telco customer of ours, AT&T. Think about a telco and the regulatory requirements of CDRs, call detail records. In the United States, a telco is required to keep seven years of call detail records for every one of us. That’s a lot of data, right? Why is it required to do that? Well, there are law enforcement and government needs to access that data. So, does it make sense to keep seven years of CDR data in a high-performance database? No. However, when you get that subpoena, does it make sense to access that data and join it with more recent data in a very specific timeline so that you don’t find yourself in court? Absolutely. That’s one good example of a company using Vertica both for advanced analytics on high-performance data, as well as archived data.
Another great example is a gaming company. Think about the right to be forgotten, GDPR [General Data Protection Regulation]. We basically own the gaming company space. If you look at the gaming industry, it’s almost all powered by Vertica. I’d say that the only industry that has more Vertica than gaming is probably Ad Tech and all those personalized ads that follow you around the web without ever giving up. We apologize for that, but it’s probably still better than constantly getting ads for things you aren’t interested in. That ad-tech is all run on Vertica too, but gaming, everything you do on Words with Friends or Wordfeud is recorded and kept. But companies are now required to honor a right to be forgotten request under GDPR.
Again, that’s archive data. You also must keep data that is real-time. How is she playing? Does it look like she’s going to buy something? What offer can we give her? You need to be able to unify that data and respond to the right to be forgotten within a legal time limit.
The same thing is true for the Ag Tech companies looking for time series trends and anomalies. How does that map to seasonality? Is that geographically distributed?
We are talking about companies like Taboola, The Trade Desk, AT&T, Zynga, that are often dealing with petabytes of data and need analytical flexibility.
And I think I’d like to leave with one final comment. The key is to think about and have some sympathy for the data supply chain optimization team. You’ve got one community of data scientists and one of business analysts, and they say, “Look, I’ve got to get my job done. I need this. I issue my query, and I need the answer, and I need it now. By the way, he wants the same analysis, but he prefers using Python. He wants a regression analysis and wants to do it with Jupyter. She wants it with SQL. They want to use Tableau and do geographic analysis on that data.” But now think to the back end, optimizing the data supply chain. If I’m the IT person, am I responsible for all that? Do I continuously have to copy, reformat, put this data here, that data there? Is it synced? Oh, did I update that?’
I don’t want to have to do that. I need to meet the demands at petabyte scale of all these users. But I also need to do it with an optimized data supply chain. And that’s what Vertica unified analytics warehouse delivers.
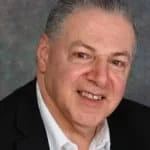
Salvatore Salamone is a physicist by training who has been writing about science and information technology for more than 30 years. During that time, he has been a senior or executive editor at many industry-leading publications including High Technology, Network World, Byte Magazine, Data Communications, LAN Times, InternetWeek, Bio-IT World, and Lightwave, The Journal of Fiber Optics. He also is the author of three business technology books.