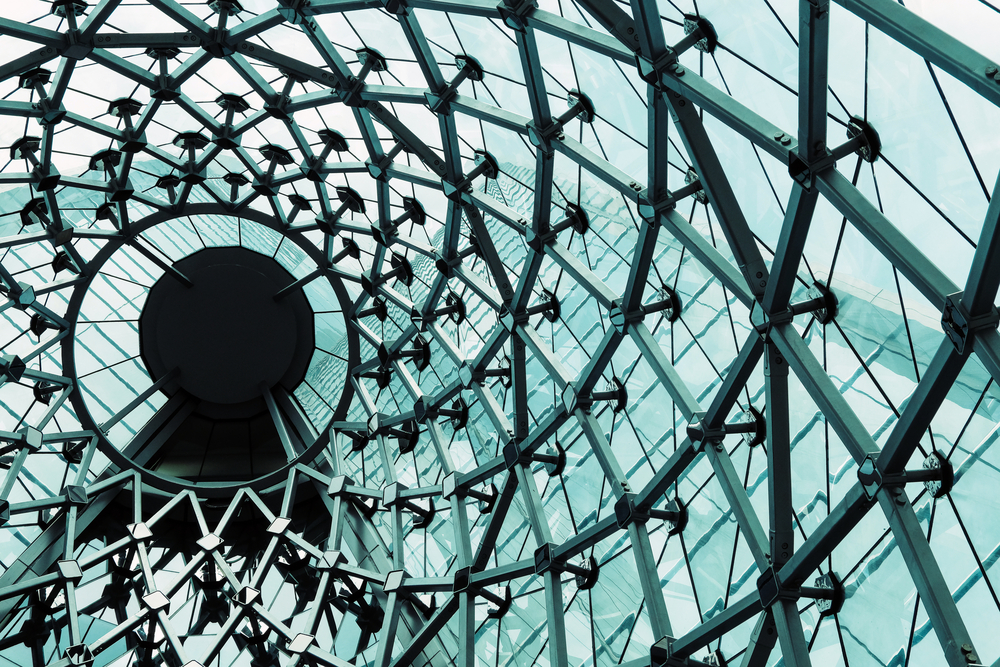
The limitations of data lakes could prevent enterprises from overcoming challenges in disparate systems and ever-evolving data demands.
In today’s dynamic business landscape, organizations strive for agility, competitiveness, and efficiency by leveraging real-time insights from actively streaming or continuously updated data sources. Real-time data is like having a live feed of your business’s heartbeat. It allows you to make decisions not based on yesterday’s news but on the unfolding story of the present. Imagine how we upgraded from a map to a GPS: real-time data analytics processes and analyzes data the very moment it becomes available, empowering businesses to make timely and informed decisions in a rapidly changing environment.
Understanding data lakes
A Data Lake is a solution for managing and storing vast, diverse amounts of raw, structured, semi-structured, and unstructured data by providing a centralized repository. To maintain the data lake, data architects and data scientists work in separate teams. Data architects are often closer to IT and focus on structure, while data scientists are generally more closely connected to business objectives. This can lead to siloed thinking and a tendency to view a data lake solely as a structural issue.
Data lakes demonstrate proficiency in managing batch processing and storing vast amounts of raw data. However, they raise major security concerns because they contain many different types of data, some of which may be sensitive or have compliance requirements. Due to the absence of database tables, permissions are more fluid and difficult to set up, and must be based on specific objects or metadata definitions. Additionally, they might not be the optimal choice for scenarios that demand real-time processing and insights. Real-time data processing, above all, allows the organization to make customer-centric decisions and play a vital role in gaining trust and loyalty.
See also: The Four Kinds of Software to Process Streaming Data in Real Time
The limitations of data lakes
Let’s delve into the key objectives that organizations aim to achieve with real-time insights, which data lakes may not fully address.
- Immediate insights: Data Lakes often relies on batch processing, which introduces latency in ingesting, processing, and analyzing data. Real-time scenarios demand quicker responses than batch processing can provide.
- Enhanced responsiveness: Data Lakes typically follow a schema-on-read approach, allowing flexibility in data structure. However, this flexibility can lead to challenges in enforcing real-time data schemas, which are crucial for immediate processing. This hurts business responses to rapidly changing conditions, market trends, and customer behaviors, gaining a competitive edge.
- Complexity in data governance: Real-time data requires stringent governance for quality and accuracy. Data Lakes, designed for flexibility, may struggle with enforcing real-time governance policies, potentially leading to issues in data consistency.
- Limited support for streaming data: Traditional Data Lakes are not optimized for handling streaming data, which is essential for real-time scenarios. Streaming architectures or dedicated real-time systems are better suited for ingesting and processing data as it arrives.
- Personalized experiences: Real-time data enables the delivery of personalized and targeted experiences to customers, enhancing satisfaction and engagement.
- Accurate analytics: Real-time systems provide up-to-the-minute data for analytics, ensuring that insights are based on the latest information, which is crucial for strategic planning.
- Adaptability: Businesses can quickly adapt to market changes, customer preferences, and external factors by having real-time insights to guide strategic decisions.
Data lakes in the real world
Let us look at a few real-life scenarios where real-time analysis plays a vital role:
Fraud detection and prevention
Real-time systems can instantly analyze transactions or user activities, identifying and preventing fraudulent activities in real-time. Traditional Data Lakes might have delays in processing and analyzing large volumes of data, making them less effective for immediate fraud detection.
Dynamic pricing in e-commerce
Real-time systems can adjust product prices in real-time based on demand, competitor pricing, and other factors. Data Lakes, while valuable for historical analysis, may not provide the speed required for dynamic pricing adjustments in rapidly changing market conditions.
Supply chain optimization
Real-time systems enable organizations to track and optimize supply chain activities as they happen, helping to prevent disruptions and improve efficiency. Data Lakes may not provide the timely insights needed to react to supply chain events in real-time.
IoT monitoring and control
Real-time systems are crucial for monitoring and controlling IoT devices, ensuring immediate responses to sensor data and maintaining optimal device performance. Data Lakes are more suitable for long-term storage and analysis, but real-time needs are better met by systems designed for IoT data streams.
Customer interaction in online services
Real-time systems allow businesses to personalize user experiences in real-time based on user behavior. While Data Lakes can store vast amounts of customer data, real-time systems are more effective for delivering instantaneous personalized content.
Leverage the strengths of data lakes to help harness data
While data lakes offer the advantage of storing vast amounts of data in its original format, their inability to handle non-standard formats and curate data for specific purposes presents a significant challenge. On the other hand, digital transformation is accelerating, leveraging real-time insights is crucial for staying competitive. Embracing data-driven decisions is not just a choice; it is an urgent necessity for ensuring long-term organizational success. By harnessing the power of data, organizations can handle challenges and thrive in a competitive environment.
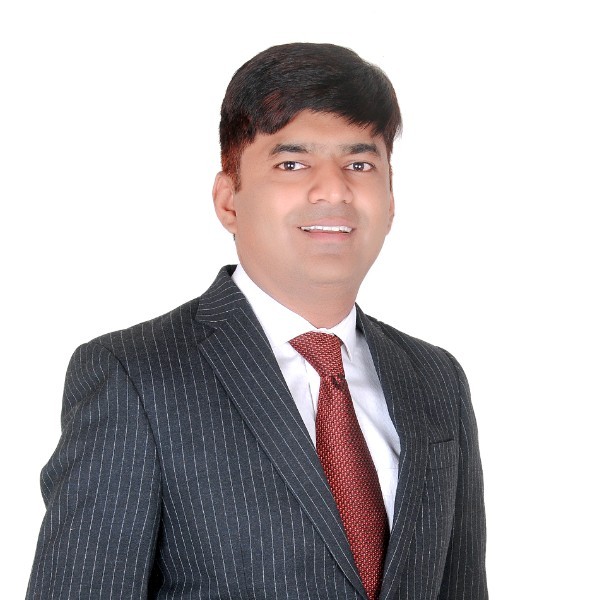
Sapnesh Agrawal is director of engineering at Gathr Data Inc, the world’s first and only data to outcome platform. With a career spanning over 2 decades, he is a seasoned architect of progress within the dynamic realm of Software products, having a journey through cutting-edge technology and visionary leadership. He has curated high-performing teams to bring visions to life. His professional tapestry weaves through multiple domains, ranging from the dynamic landscapes of data analytics and no-code application development platforms, and the rigor of Investment Banks, to the mission critical needs of Lawful Interception.