Data downtime creates a downward spiral of company culture, stopping companies from achieving the degree of data-driven decision-making that they aspire to.
In decades past, downtime was an accepted part of using the Internet—remember Twitter’s infamous “fail whale”? Today, the standards have intensified, and downtime has evolved into not just customer-facing applications and services but internal ones, too. As more companies tally up the impact of downtime on their internal tooling—everything they use to be a data-driven organization—they started to put a new focus on data downtime.
The term was coined by Monte Carlo, a data observability platform, based on the experiences of its CEO, Barr Moses, while working at Gainsight. Moses writes: “Data downtime refers to periods of time when your data is partial, erroneous, missing, or otherwise inaccurate. It is highly costly for data-driven organizations today and affects almost every team, yet it is typically addressed on ad-hoc basis and in a reactive manner.”
Data downtime lags behind customer-facing downtime by years—it’s still in its own “fail whale” state, but that’s changing quickly. In the past, a company might have established an internal availability SLA for its data team to ensure that internal tooling stays online, but teams simply waited out the issue. But today, more companies are treating it as an on-fire issue, and those involved in observability need to update not just their tooling but their way of thinking.
See also: What Makes Cloud Observability Critical and Different?
An example of data downtime
Every Monday morning, the VP of Product sits down at their desk and opens up a few dashboards ahead of their team’s weekly strategy meeting. They’re looking at new user growth, daily active users, attribution metrics, demographic information from new users, in-product usage behavior, and more. These dashboards were designed in sync with the data team to give the product team all the information they need to improve onboarding flows, make UI/UX decisions, and more.
But the VP sees that some of the data doesn’t look right—they expected better new user retention given the interactive product tour feature they shipped last week, and everyone is focused on the niche features, not the core functionality their company is known for.
The VP doesn’t trust the data they’re seeing more than they believe there could be something wrong with the application—or their previous decisions. They think it’s much more likely to be an issue with the data pipeline, which means they set off a chain of painful events, roping in the data engineering, analytics, and product development teams to figure out what’s going wrong. Issues in the frontend code? Problems with ingesting data into the data lake? Analytics tools coming to incorrect conclusions? Data downtime is already affecting not just availability but, more importantly, company culture.
See also: The Role of AIOps in Continuous Availability
The unexpected harms of data downtime
Regardless of the outcome from the VP of Product’s frantic email about the data issues, it’s going to happen again. Maybe not next Monday, but it’s an inevitability. And that’s the most problematic aspect of data downtime: an unbreakable cycle of pesky bug fixes. Instead of building out the product and improving the customer experience, an organization’s engineering talent is focused on improving the availability of data.
Why this cycle? Stakeholders have lost their trust in the data. If something doesn’t go their way—if they’re on track to miss a KPI or see a sudden unexpected (and negative) spike, it must be because of a fault in the data. Maybe the dataset is corrupted, or the averaged results aren’t accurate because of gaps in data availability. They’ll start to wonder: This chart was wrong last week, but the data science team promised me they fixed it. But if that chart could be so wrong, how do I know these charts aren’t wrong now, too?
These harms stop companies from achieving the degree of data-driven decision-making that they all seem to aspire to. They’ll fall back on making gut decisions, unfounded by reality, and have a convenient excuse when it doesn’t go their way. Data downtime creates a downward spiral of company culture, where dashboards become a liability. Moses of Monte Carlo even recounted talking to a CEO who walked around the office, putting sticky notes on every monitor they believed showed erroneous data.
Heading toward a solution: data observability
There are more ways than ever for a company’s data to crash, from more disparate data sources, bigger teams, and astoundingly sophisticated data pipelines. And because of the impacts—both in time and company culture—of data downtime, reducing it is actually a customer-facing directive.
Data observability is one growing solution. These platforms don’t just collect metrics—they give teams transparency into every part of their data pipelines so that they can explore and resolve the unknown unknowns within their infrastructure—all of the unexpected ways that even well-architected systems can fail.
Commercial platforms include Snowplow, Monte Carlo, and Honeycomb, while open-source projects like OpenMetrics and OpenTelemetry aim to develop some of the same functionality to companies that choose to build their own pipelines using Prometheus/Grafana, the ELK stack, Jaeger, and more.
Relying on the fail whale won’t cut it any more for internal tooling. For those who recognize the impact these internal tools have on their customer experience and want to avoid the cultural clashes that emerge from data downtime, they’ll prioritize the health of these pipelines in 2022 and beyond.
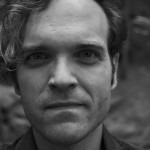
Joel Hans is a copywriter and technical content creator for open source, B2B, and SaaS companies at Commit Copy, bringing experience in infrastructure monitoring, time-series databases, blockchain, streaming analytics, and more. Find him on Twitter @joelhans.