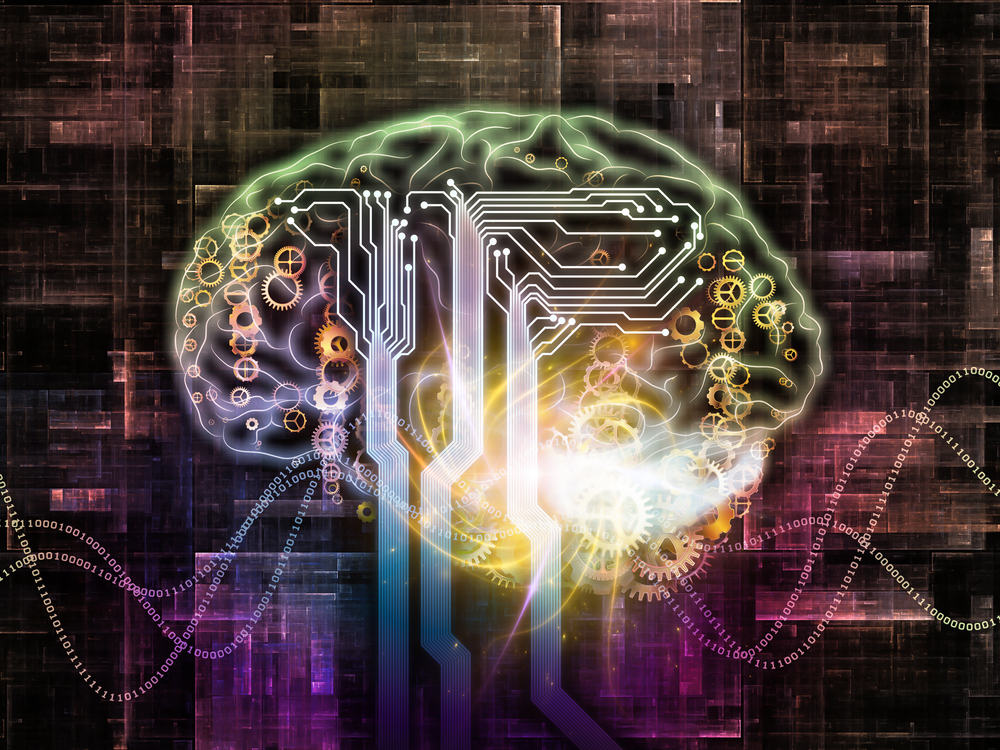
Today, businesses are drowning in information that can lead to new opportunities and the next breakthrough strategy, but they are still starved for knowledge. The need to effectively generate insights and constantly look for ways to innovate the product has propelled decision-makers to rely on AI and ML technologies. Forbes predicts that AI-powered software may reach a market value of $125 billion by 2025.
The move to the cloud has made AI and ML even more accessible now, and businesses are investing heavily in AI/ML initiatives to automate processes, improvise strategies, predict the future and uncover insights among their billion-scale datasets. Such a well-informed and data-driven approach helps reduce costs, improve efficiency, augment customer experiences and obtain a competitive edge.
AI/ML in cloud computing makes businesses more efficient, effective and profitable. Let’s explore the impact of AI/ML on a business landscape.
See also: AI Workloads Need Purpose-Built Infrastructure
Intelligent Automation
Businesses leverage AI and ML to automate various repetitive and time-consuming data engineering and analytics tasks. By integrating cloud-based automation into their workflow, they can streamline data cleaning, transformation and modeling processes.
With advanced algorithms, machine learning capabilities or robotic process automation (RPA), organizations can automate tasks like data entry, inventory management, customer support, etc., and release their employees from redundant workloads, enabling them to focus on more value-adding strategic initiatives. This smart automation saves time, minimizes errors, improves accuracy and drives efficiency.
Elasticity and Scalability
AI in cloud computing offers extensive data management capabilities with the flexibility to build a seamless environment. By leveraging the elasticity of cloud technology, AI/ML models have access to unlimited data and can scale up and down depending on workload. It helps businesses analyze billion-scale datasets to improve business performance without having to invest in additional hardware and infrastructure, even when the number of users increases.
Predictive Analytics for Informed Decisions
Many organizations use multiple cloud environments and deal with siloed infrastructure monitoring, management and troubleshooting solutions. As a result, they often overlook the correlating information across the infrastructure, leading to missed insights.
Predictive analytics uses AI/ML in cloud computing to improve processes and analyze historical data like customer buying patterns, weather patterns or gas price fluctuations. Regardless of the intricacy of the cloud network, syncing real-time data with advanced algorithms unlocks actionable insights. Identifying patterns and trends helps plan inventory, optimize delivery and improve forecast accuracy.
Improved Customer Experience with Personalized Marketing Initiatives
Businesses can use AI/ML-based predictive analytics to personalize customer offerings by analyzing their buying behavior and preferences, optimizing marketing strategies and improving customer retention.
The journey to reinforcing impeccable and personalized customer experiences begins by identifying the touchpoints of customers’ interaction with employees from multiple channels. Once they identify the traffic, AI-powered virtual assistants and chatbots can provide round-the-clock support and faster response times.
Fraud Detection and Cybersecurity
The complexity and intensity of cyberattacks have been growing for some time now. AI and machine learning can augment security analysis when battling automated, well-orchestrated cyberattacks and breach attempts. No wonder, more businesses are investing substantially in AI and ML-based endpoint security control applications to identify the probable areas of vulnerability in their systems or processes. Based on recent market research, Gartner predicts $188.3B will be spent on Information Security and Risk Management in 2023, increasing by a CAGR of 11.3%.
To combat security risks at all levels, AI/ML in cloud computing performs real-time scans of enterprise-wide processes to derive risk areas based on previous behavioral patterns, login time, geolocation and many other variables. This monitoring can flag suspicious behavior, detect potential threats and prevent fraud before it occurs, improving endpoint security.
See also: Cloud Security: A Primer
Competitive Advantage
Analyzing data manually and guessing about market disruption isn’t the right way to predict today’s revenue shift. Businesses need to be able to analyze cloud-scale data in sub-seconds to get ahead in the market.
By harnessing the power of AI/ML in cloud computing, decision-makers can boost efficiency through automation, detect fraudulent patterns, use customer insights to make intelligent decisions and uncover hidden opportunities to gain a competitive edge in the market. It helps churn the algorithms and surf the cloud-scale data to analyze the next trend and selling point, target the correct leads and audience and optimize where productivity should be partially increased or decreased.
System Integration Challenges
With all the speed, efficiencies and innovations that come with AI/ML in cloud computing, there are some challenges in system integration . Since the models highly rely on data quality and accuracy, addressing these concerns beforehand is essential.
Limited Knowledge
Cloud-based AI is a better alternative to traditional systems, but the real concern for organizations is to find and hire the right talent. The success of cloud AI hinges on the professional expertise and skills required to increase production, manage resources, understand customer behavior and react to market conditions accordingly. Unfortunately, only a limited number of professionals with the required qualifications – who understand the potential of AI/ML on the cloud – currently work in the industry.
Cost Overruns
Cloud-based AI/ML systems are required in various sectors, such as healthcare deployment, asteroid tracking, tracing of cosmic bodies, etc., each demanding an ever-increasing number of GPUs and cores to work efficiently, or sometimes they need a supercomputer’s computing power, which is not cheap.
Not every small or large organization can spare resources and investments for cloud-based AI/ML systems, especially with an increase in the inflow of ever-growing data and complex algorithms.
Data Bias
The efficiency of cloud-based AI systems depends on the data used to train them. Suppose the data used to train the system is insufficient in terms of the population it is meant to serve. In that case, it can make biased decisions or recommendations, bringing more harm to an organization than good. For example, a facial recognition algorithm trained on data sets of a particular ethnicity can get incorrect or biased results when used on individuals of different races.
Data Security
Organizations move massive amounts of data to the cloud when using cloud-based AI/ML systems. The data transferred to the cloud might be generated from millions of users, and there is a possibility that this data can be used for fraudulent purposes.
For example, suppose one million people (about the population of Delaware) in a city use bank services, and due to a cyber-attack, the account details of all the users fall into the hands of everyone on the dark web. This data includes account number, debit card/credit card information, account balance, etc.
The attackers from the dark web can use this data to drain bank accounts. To make matters worse, if the bank has several branches nationwide, data flows in from everywhere. There is undoubtedly a possibility of data leakage, and attackers can utilize leaked data to get credentials or log into corporate systems to deploy malware.
See also: Why the Need for a Post-Modern Data Stack?
Stay Ahead of the Curve with the Right Technology Solutions
In this AI-powered digital age, organizations need modern technology that can help overcome all the above-mentioned challenges. Without necessarily needing the comprehensive knowledge of any underlying analytics tool, business users can harness AI/ML-based OLAP on the cloud, leveraging pre-processed data models.
Optimizing resource consumption with price-performant queries and a distributed, elastic architecture can reduce costs. Users can analyze as much data as they want or need to reduce the chances of bias in results. With a universal semantic layer, they can democratize data access, standardize metrics and implement cloud-native security architecture with role-based access controls. All in all, they need a highly scalable, flexible and inclusive analytics stack to make the most of their AI/ML investments in the cloud.
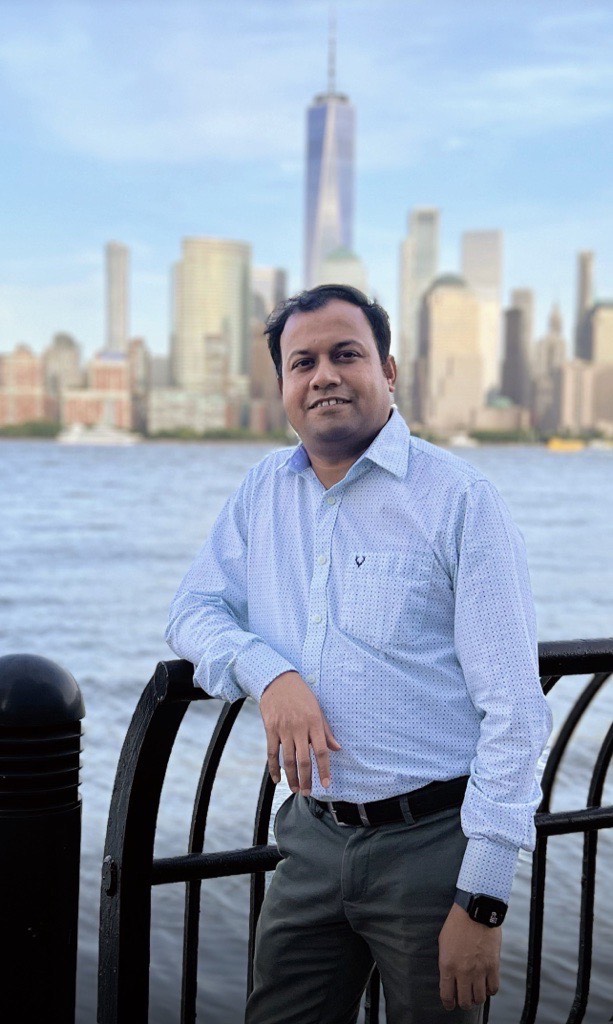
Sajal Rastogi is the Director of Technology at Kyvos Insights. Prior to working with Kyvos, he has worked as an Enterprise Architect with exposure to building large scale distributed and scalable solutions using Service Oriented architecture and BigData technologies. he holds an experience in establishing overall architectural view, identifying major system interfaces, design and develop enterprise-wide reusable software and modules for multiple products. Along with managing the core engineering for backend teams for more than 10 years now, he has also implemented a big part of the product backend. He had taken initiatives for implementing software engineering processes for the company like Agile, Automation and CI. With more than 8 years of experience in Big Data related technologies, he is very passionate about his work, learning new technologies and solving complex problems. He is a strong leader who believes in leading by example.