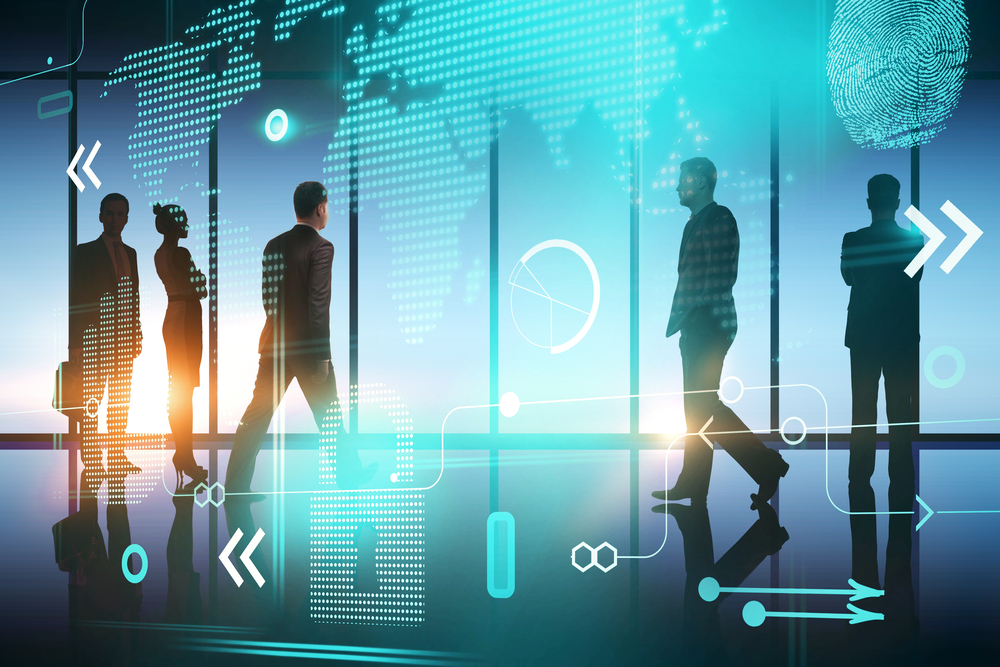
Data democratization is a multi-faceted initiative frequently led by creating a systems architecture that allows for the actual or virtual pooling of data. Then there are countless adjacent areas to adjust such as security, privacy, governance, and performance to name a few. It requires a sizeable investment and commitment to change the organizational culture around data usage and build a sustainable infrastructure–at enormous scale. There are many conversations around the computing and data storage scale required to handle workloads that might now access petabytes of data instead of terabytes. Now the user-count must scale along with the number of users expecting answers returned in 1 to 3 seconds. Considerations of scale have just grown exponentially.
The Gartner Data & Analytics Summit gathers leading industry analysts, major technology providers, and data strategists from all industry and government sectors. It’s where everyone goes to take the pulse of the market. Altair’s SVP of data analytics, Mark Do Couto, and Ingo Mierswa, SVP of product development shared their read on what customers are seeking to accomplish with advanced analytics and machine learning. Cloud Data Insights (CDI) thought that talking to these executives who bring a record of HPC innovation together with a platform for radically opening data analytics to a broader circle of users would lead to some unique perspectives.
CDI: How have your customers’ needs or attitudes changed given current economic conditions and pandemic-driven digital transformation?
Mark: As businesses continue to expand their operations and move toward digitalization, software purchases have become increasingly important. However, customers are no longer satisfied with simply purchasing software for the sake of having it. Instead, they are now focused on ROI and are leveraging data insights to make informed business decisions. This has led to a shift in emphasizing the value of software and the benefits that it can provide to businesses.
As businesses generate more and more data, it has become essential to have the right tools and software in place to make sense of it all. They are looking for ways to enable more people in the enterprise to take advantage of data by encouraging the citizen data scientist who does not need to know how to program or code to gain insights from data. By leveraging these insights, businesses can make more informed decisions and take proactive steps to improve their operations. Ultimately, this has led to a more data-driven approach to business decision-making.
CDI: How are you responding? Have you adjusted your product strategy or roadmap? Or your approach to sales?
Mark: At Altair, we continue to focus on building tools to facilitate this shift. The data analytics team is working on best-in-class data solutions and becoming trusted partners with our customers.
Especially post-pandemic, our customers want as much value from their vendors as possible. So we have a unique licensing model which allows them to have full access to Altair RapidMiner, our data analytics and AI platform, while also taking advantage of our more than 150 individual products.
See also: Operationalizing Data and Analytics
CDI: What are the main technical challenges your customers face? Does this mark a change from a year ago?
Ingo: Our customers appreciate the massive acceleration and value that can be achieved with data analytics in general, and machine learning (ML) and artificial intelligence (AI) in particular. But advanced analytics can be daunting and often organizations do not know where to start or they have high-value use cases but not the skills in-house to drive the adoption of ML and AI to solve those.
So while this is related to technical challenges as a result of the complexity of this field, it is as much an organizational or people challenge. How can we empower more people to automate decisions? How can we train employees to ask questions they never dared to ask? We have all the technical solutions for doing so. Just consider the recent breakthroughs of generative AI and large language models. But we need to empower anybody to move fast so that competitors are not getting too much of a competitive edge.
We call this Frictionless AI. Moving to the insights you want faster than ever. To remove all the friction points – technical, cultural, or people-related – customers need to invest in the right platform, their people, and into bringing together their data, their people, and their expertise. Only then can advanced analytics be used at its fullest potential.
The complexity of AI did not change much compared to a year ago. But what did change is the competitive pressure and organizations realize very quickly that they need to move fast to keep up or, even better, lead ahead of their competition.
See also: Frictionless AI: The Key to Delivering on AI’s Promise
CDI: Which emerging technology or business trend do you think will have the most impact on your company?
Ingo: For most team members, generative AI and large language models will have the biggest day-to-day impact. Repetitive tasks will be solved faster than ever and the combination of human and machine knowledge and creativity will deliver larger impact faster than ever. We will keep empowering anybody to make use of these and other technologies in the easiest way possible.
The other exciting area is what some people call Composite AI. This means that we will see a convergence of machine learning with natural language processing, simulation, and optimization among others. This brings advanced analytics closer to the business problems because machine learning models become the full solution, not just a part of one. The convergence of these fields will help our customers get the most out of a composite solution.
CDI: One of our observations is the sharp increase in the adoption of real-time analytics. First came the emphasis on streaming data where real-time ingestion was an obvious requirement. But how quickly could that real-time data be utilized? Also in real-time? What are you seeing among your customers?
Mark: That was one of the reasons Altair acquired Data Watch, which was focused on real-time streaming data and real-time data preparation. Those naturally would eventually lead to real-time utlization and then real-time analytics.
Ingo: At the end of the day, it’s the customers who have really driven the real-time use case. The faster a customer can get a response, whether it’s an approval for a credit card, or to make a payment at the register to buy a laptop, the better the customer experience is, the faster a business can transact.
Now, I think it’s important that we still review that data and make sure the models are being augmented with the real-time data coming, but I think the ability to leverage that real-time data with the models that are being built to help make those business decisions are what customers are looking for.
CDI: How does the need for human review or validation of models affect automation, at least in the case of ML-enriched transactions?
Ingo: This maybe is connected to the maturity of machine learning overall. I think many people are still wondering where’s the value generated from machine learning models? They believe that if the models could reveal one super-smart piece of advice that billions of dollars will emerge. That’s actually not the biggest value of rich machine learning–it’s really those use cases with tons of “small” decisions, which barely seem to warrant any attention. Yes, you could have hundreds of people observing every single transaction that might look suspicious, but it’s far too expensive to have a human do this. When you automate decisions, it’s not the one multibillion dollar decision that counts, but it may be billions of small decisions where the value of ML lies. This might also be one consideration to weigh the value of streaming data.
See also: The Hyper-automation Dilemma: Reconciling Employee Needs While Accelerating Growth
CDI: Streaming data and “small” decisions bring to mind IoT and edge data. How much have these brought customers to you?
Ingo: It’s probably 40% plus and most of the new use cases are actually somewhere connected to IoT sensor data from, for example, from production processes. The usage of this data has gone beyond the predictive maintenance use case. You could also predict the quality of the product you are currently producing. This product isn’t making our quality thresholds, so we probably won’t be able to sell it. Why am I actually still putting energy into this? Maybe I should stop the production process until we improve our yield? You can start putting sensors everywhere and you generate lot of data for this one particular use case. But once the data is available people get more and more creative about other questions they can ask and develop new use cases for the same data. Automotive is one area that we are seeing this phenomenon as lot, and of course with banking and financial services. On-line banking is bringing a lot of existing data into new use cases.
CDI: You’re describing a situation where an organization goes from having one question and a limited set of data points to having so many data points that you can’t grasp the scope of the data but eventually, driven by business people’s and data people’s curiosity, you can weave them together to make much more complex decisions.
Ingo: That does require a bit of a cultural change and some investment into a platform if you ever want to bring all these data points together for more than a one-off project.
So what do we need to do differently? Maybe empower more people to actually be able to ask their own new questions of data? You’ll find you don’t need to look hard for new use cases, because someone wakes up every single morning and realizes,” wait a second, why are we not using machine learning for this scenario, too. It really transforms how you’re actually solving problems. Experiencing this happen for an organization is for me, personally on of the most exciting things.
CDI: Are you suggesting that if you have the right platform to bring data together and provide good self-service inquiry or exploration, people will flock to it?
Ingo: It may sound a little weird for a platform provider to say this, but I don’t think it’s the platform. So fantastic platforms or a fantastic piece of technology, which empowers more people is only half of the equation because that’s not going to change how your employees think about data. The technical solution has to be combined with upskilling your employees for example, making sure that people understand the basic concepts of data science and machine learning. Do they need to know how to build a large language model? No. But would it be useful to know what kinds of use cases can be solved with this zoo of different technologies. And can we bring people to a point where they can translate between a business problem and an analytical approach.
Our customers still surprise us by coming up with use cases which our technology was not intended for.
Elisabeth Strenger is a Senior Technology Writer at CDInsights.ai.