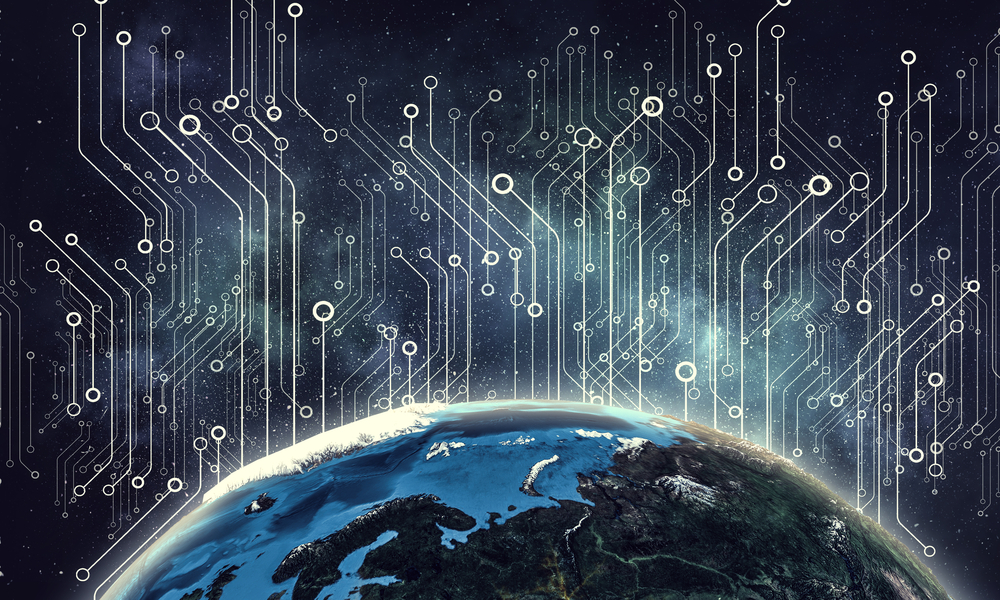
Companies are gripped in the worst neck-in-neck race of all—adopting new technology faster than cybercriminals can. If this statement seems dramatic, consider this: cybercrime is expected to cost upwards of $8 trillion annually by the end of 2023. The same tech that’s enabling businesses to handle massive data, create consistent and accessible connections, and enable remote operations is also giving threat actors ways to overcome traditional security procedures.
Thanks to this sophistication, organizations are finding their own innovative security measures, and at the front lines of this stands artificial intelligence and machine learning. Thanks to an enlightening webinar on the practical applications of AI/ML in security monitoring and analytics from Elango Balusamy, Co-founder & CTO of SquareShift Technologies, we can understand how.
See also: Addressing Cloud Native Security Risks in an Evolving Landscape
The Evolving Landscape of Cybersecurity Threats
The state of cybersecurity is constantly in flux in most categories except for one — how fast threats are growing. The current landscape has seen explosive growth in the type, duration, and frequency of incidents, leaving companies barely any time to breathe before the next big threat comes around the corner.
- CrowdStrike’s 2023 Global Threat Report found a 95% increase in cloud exploitation, with more threat actors demonstrating cloud-conscious behavior. Threat actors continue to exploit vulnerabilities in architectural weaknesses.
- Trend Micro’s 2023 MidYear Cybersecurity Threat Report identified 14 new ransomware families in 2023 and reiterated that threat actors are using artificial intelligence to streamline and scale cybercrime activities.
- Kroll’s xQ2 2023 Threat Landscape Report highlights supply chain vulnerabilities due to fast adaptation from threat actors. Even current best practices like multi-factor authentication may not stop cybersecurity incidents of tomorrow.
- A 2022 study from IBM found that breaches where remote work was a factor cost nearly $1 million more than incidents where remote work wasn’t a factor.
What do we take from this? Companies need help executing full-scale, consistent, and thorough cybersecurity policies, of course, but even more than that. They need cybersecurity policies that scale and adapt—and quickly.
The Promise of AI and ML in Security
Traditional security approaches, while effective to a certain extent, are increasingly being supplemented or replaced by the power of Artificial Intelligence (AI) and Machine Learning (ML). This section will delve into a comparative analysis, highlighting the key distinctions between AI/ML-driven security and traditional methods.
Traditional Security Methods
Traditional security measures rely on rule-based systems, signature-based detection, and known threat indicators. While these methods have been the cornerstone of cybersecurity for decades, they have notable limitations:
- Reactivity: Traditional approaches are often reactive, relying on known attack patterns and signatures. They struggle to identify novel or zero-day threats that lack predefined signatures.
- High False Positives: The reliance on rule-based systems can lead to increased false positives, inundating security teams with alerts that require manual investigation and often result in genuine threats being overlooked.
- Limited Scalability: As the threat landscape expands in complexity and volume, traditional security tools struggle to scale effectively. They can become overwhelmed by the sheer volume of data generated by modern networks and applications.
- Lack of Context: Traditional methods often lack the ability to analyze contextual information, making it challenging to differentiate between normal network behavior and suspicious activities.
- Human Resource Intensive: These approaches require significant human intervention for threat analysis and response, which can strain security teams and slow down incident resolution.
AI/ML-Driven Security
AI and ML technologies have the potential to address many of the limitations of traditional security methods:
- Proactivity: AI/ML systems can proactively identify and respond to emerging threats by continuously learning from data patterns. They excel in detecting subtle anomalies and previously unseen attack vectors.
- Reduced False Positives: Machine learning models can be trained to reduce false positives by learning to distinguish between benign and malicious behavior over time. This reduces alert fatigue and allows security teams to focus on genuine threats.
- Scalability: AI and ML can process vast amounts of data at high speed, making them well-suited for modern, data-rich environments. They can handle the scalability demands of today’s networks and applications.
- Contextual Analysis: AI/ML systems excel at contextual analysis, considering factors such as user behavior, device attributes, and network context to make more accurate determinations of threats.
- Automation and Augmentation: These technologies can automate routine security tasks and augment human decision-making. They enable security teams to respond faster and more efficiently to incidents.
Real-World Use Cases
According to Balusamy, there are several excellent use cases for AI:
- Threat detection: These programs can predict threats from unusual patterns and take a predictive analysis approach. Add real-time monitoring and natural language processing to help provide the next steps, and this could be a game changer for cybersecurity teams.
- User behavioral analysis: Previous cybersecurity relied on rule-based analysis. User behavior analysis helps reduce false positives by learning from the baseline of normal user activity.
- Log analysis and event correlation: This offers dynamic risk-based prioritization value, giving teams more input for what alerts are the most important. Because a single event is not always enough to indicate that something has happened, context for correlation is a critical security layer.
- Security Orchestration and Automation Response (SOAR): Building in automation and orchestration helps ensure continuous compliance.
How is the industry implementing these tools?
Balusamy sees several industry trends happening when implementing AI in a cybersecurity strategy. Endpoint security is one. Advanced malware threat detection for Zero-Day threats is another. In both of these cases, AI/ML based threat detection is helping companies respond more quickly to previously unknown threats and prevent some of the most common threats (happening at endpoints).
He also sees AI/ML as an extension of AIOps. Automation of operational processes gives companies a stronger security posture. Within this, an easy ROI is threat detection and noise reduction. Companies experiencing alert fatigue can now identify and respond to what is top priority and reduce the number of false positives and missed alerts.
See also: How to Make the Invisible Serverless Threat Landscape Visible
Building stronger security
AI/ML offers a proactive, scalable, and context-aware approach to threat detection and response. Companies can combine the strengths of both traditional security and AI/ML supported measures to help ensure a robust response to threats that can change overnight.
Be sure to view the full webinar for more details into building AI/ML into your cybersecurity solution.
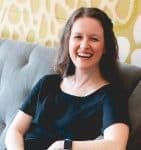
Elizabeth Wallace is a Nashville-based freelance writer with a soft spot for data science and AI and a background in linguistics. She spent 13 years teaching language in higher ed and now helps startups and other organizations explain – clearly – what it is they do.