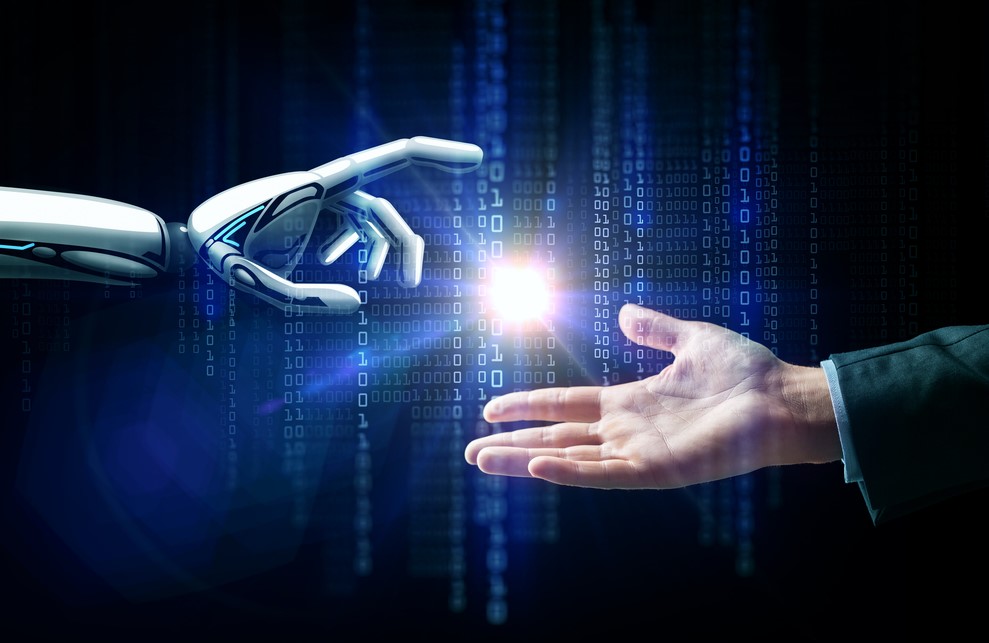
In the wake of the recent attention on large language models with Chat-GPT, LLaMA, and others, the AI space race has been kicked into overdrive. These models demonstrate tangible, practical use cases currently centered around search that seemingly provide immediate value for users.
Recent concerns from OpenAI’s Chief Scientist about the availability of these models in an open-source ecosystem, combined with ongoing hallucinations from these newly available generative AI models, underscore the immaturity of the AI ecosystem and the caveat to seemingly realized value from AI model output. While ROI from AI is typically quantified via cost savings, revenue generation, insight discovery, etc., it’s important to keep in mind that artificially intelligent applications need to be leveraged with a system of Responsible AI checks and balances. Mitigating bias, establishing trust and transparency in data lineage, and creating processes to monitor the outcome of model inference ensure more scalable long-term benefits from AI.
We offer this prescriptive guide here for companies who are starting or already have started their AI journey to establish Responsible AI principles and practices.
See also: 6Q4: Responsible AI Institute’s Seth Dobrin on Attesting that Your App Does No Harm
State of the Market
Businesses see AI as a gateway to strategic advantage in the market and are looking for opportunities to institute this technology into their existing workflows. BCG and MIT conducted a survey of global business leaders and found that 83% of executives view AI as an immediate strategic priority, and 75% of respondents believe AI will help them move into new businesses and ventures. AI has become a ubiquitous part of the modern analytics stack and a crucial CapEx investment to help businesses foster innovation at faster speeds.
However, despite a 163% increase in global AI investment from 2019 to 2021, projected to be $500B in 2023, over 85% of AI projects are not delivering value to businesses or consumers, namely because of challenges related to:
- Purchase Expectations (and time to value): Misled positioning in terms of the function and implementation of AI to solve a core business problem can lead to unmet expectations for ROI
- Improper Build Practices: The development process for AI can often focus too heavily on the model lifecycle vs. being human, and data centric; teams are misaligned, using different interpretations of data, and are not optimized for long-term success
- Consumption Deficiencies: Model deployment often takes too long because of 1 and 2, and as a result, tends to rush businesses to disregard the mechanism by which model output can be made understandable, trustworthy, secure, and unbiased
So a lot of AI projects – over 50% – fail to make it into a production environment and are oftentimes not scalable or responsibly considering the downstream impact.
See also: NIST: AI Bias Goes Way Beyond Data
A Responsible AI Framework
We put together this simple framework to help you institute Responsible AI practices in your organization to make sure your AI projects are successful for you and your customers:
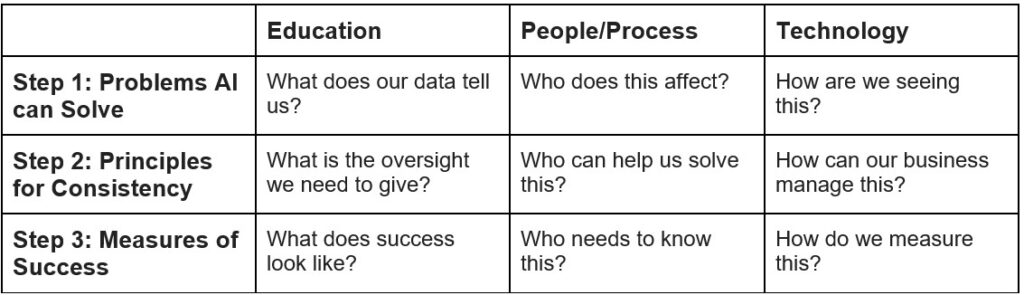
Step 1: Problems AI can Solve
Education – Understand what your operational and analytical data tell you and the capacity of AI to address the problem
People/Process – Ensure team alignment to an objective, and take a human-centered design approach to consider multiple roles, backgrounds, and perspectives
Technology – Instill effective mechanisms to measure consistently and accurately
Step 2: Principles for Consistency
Education – Give consumers insight into how, where, and for what their data is being used when AI is in the picture
People/Process – Create boards, committees, and centers of excellence to help drive similar approaches across an organization
Technology – Look for agnostic tools that can work with existing cloud and data warehouse platforms to optimize the speed of implementation, developer productivity, and compute resource spend
Step 3: Measures of Success
Education – Learn about the effectiveness of AI in the application by asking end consumers
People/Process – Create regular cadences to ensure transparency, inclusivity, and reliability of the model in addressing the core business challenge
Technology – Leverage TrustedAI platforms, MLOps, and existing BI tools to mitigate risk, model performance, and create simplistic views to measure
It’s never too early to start – creating not just an AI practice but a Responsible AI practice leads to more successful AI projects that drive business value. Companies that are implementing Responsible AI agree on the benefits:
- 50% of leaders agree their products and services have improved
- 48% of leaders have created brand differentiation
- 43% of leaders have seen an increase in innovation
Forty-one percent of RAI leaders confirmed they have realized some measurable business benefit compared to only 14% of companies less invested in RAI. – MIT Study on Responsible AI
While still in its infancy, Responsible AI has proven to be successful for businesses investing in AI, leading to innovation and alignment to other strategic objectives:
- eBay has used Responsible AI principles to educate its employees about the importance of corporate social responsibility
- Levi Strauss has used Responsible AI principles to create a consistent process for the implementation of AI across multiple business units.
- H&M has used Responsible AI principles to help measure and drive ambitions for environmental sustainability
Responsible AI practices mean a successful AI practice. A successful AI practice enables the scale of AI projects. More responsible AI means more business value.
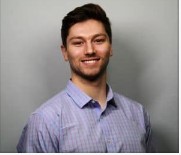
Zachariah Eslami is currently Director of Product Management – AI/ML at AtScale. He is passionate about the application of data science and AI to help solve key challenges with data, and works with customers to help them develop and realize value from AI models. Prior to his time at AtScale, Zach worked as a Speech ASR/NLP Product Manager at Rev.ai, managed a team of solution engineers at IBM, and helped to productize the IBM Watson AI core application platform. Zach currently lives in Austin, Texas, in his free time, he enjoys playing tennis, photography, and playing piano.