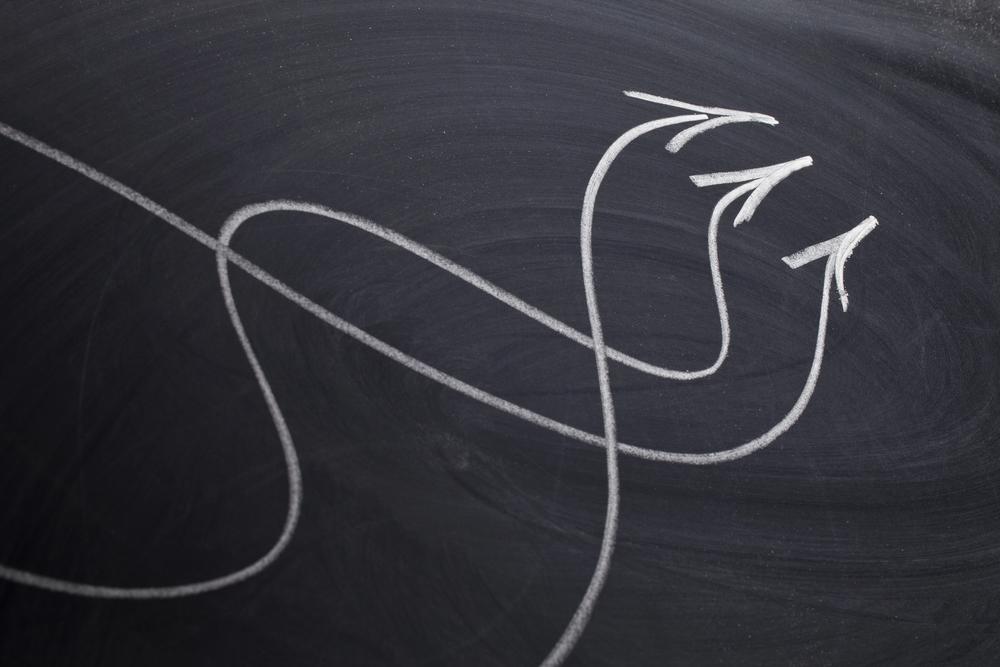
The convergence of AI, edge computing, and data-in-motion represents a powerful trend in technology that has the potential to transform various industries and enhance the capabilities of computing systems. Let’s explore each of these elements and how they intersect.
The components of the convergence
Each of these plays a role in how technology will shape the future of industry.
Artificial Intelligence (AI)
AI, of course, refers to the development of computer systems that can perform tasks that typically require human intelligence. This includes learning, reasoning, problem-solving, perception, and language understanding tasks.
AI plays a central role in the convergence by providing the intelligence needed to analyze and derive insights from data. Machine learning algorithms, deep learning models, and other AI techniques enable systems to understand patterns, make predictions, and optimize processes.
Edge Computing
Edge computing involves processing data near the source of data generation rather than relying on a centralized cloud server. It brings computational power closer to where data is produced, reducing latency and improving efficiency.
Edge computing is crucial for AI applications that require real-time processing and low latency. By processing data locally on edge devices, AI algorithms can make faster decisions, which is particularly important for applications such as autonomous vehicles, industrial automation, and augmented reality.
See also: IoT and Edge Market in Flux, Focus Turns to Industrial and Building Intelligence
Data-in-Motion
Data-in-motion refers to the streaming or real-time flow of data from one point to another. This data is actively moving and being processed as it travels between devices or systems.
Real-time data is essential for many AI applications, and data-in-motion ensures that the latest information is available for analysis. Streaming data from sensors, devices, or other sources enables AI systems to respond quickly to changing conditions, contributing to more dynamic and adaptive solutions.
The convergence: Where intelligence meets real-time processing
The convergence of AI, edge, and data-in-motion represents a holistic approach to computing where intelligent systems operate at the edge, leveraging real-time data to make decisions and optimize processes.
This convergence is particularly valuable when low latency, privacy concerns, and bandwidth limitations make centralized cloud processing less practical.
Applications of Convergence:
- Smart Cities: AI at the edge can analyze real-time data from sensors, cameras, and IoT devices to optimize traffic flow, manage energy consumption, and enhance public safety.
- Industrial IoT (IIoT): Edge AI can be applied in manufacturing plants to monitor equipment health, predict maintenance needs, and optimize production processes.
- Autonomous Vehicles: Edge computing enables AI algorithms to process sensor data in real-time, allowing vehicles to make split-second decisions without relying on distant cloud servers.
The convergence of AI, edge computing, and data-in-motion form a symbiotic relationship that enables intelligent systems to operate efficiently, respond in real time, and address the challenges associated with processing large amounts of data at the edge. This convergence has the potential to drive innovation across various industries and pave the way for more advanced and responsive applications.
Advantages of Convergence
Let’s explore how these advantages manifest in real-world applications across various sectors, highlighting the tangible benefits and illustrating the potential for innovation and efficiency gains. From smart cities to industrial IoT and autonomous vehicles, the convergence is not just a theoretical concept but a powerful catalyst for positive change across the industrial spectrum.
Convergence could enable:
- Reduced Latency and Improved Response Times: With AI algorithms operating at the edge, processing data locally allows for near-instantaneous decision-making. This is particularly crucial in applications where split-second reactions can make a substantial difference, such as autonomous vehicles navigating complex environments or real-time monitoring of critical infrastructure.
- Enhanced Privacy and Security through Local Processing: Conventional cloud-based approaches often raise data privacy and security concerns. By processing data locally at the edge, sensitive information can remain on the device, reducing the risk of unauthorized access during transit to a central server. This localized approach enhances privacy and strengthens security measures, mitigating the potential vulnerabilities associated with transmitting data over networks.
- Optimized Bandwidth Utilization by Processing Data at the Source: Instead of transmitting large volumes of raw data to centralized servers for processing, edge devices can preprocess and analyze data locally. This optimized use of bandwidth reduces network congestion and minimizes the need for continuous high-bandwidth connections, making the approach more scalable and adaptable to varying network conditions.
- Real-Time Adaptability in Dynamic Environments: In dynamic environments where conditions change rapidly, the convergence enables real-time adaptability. AI models operating at the edge can analyze incoming data streams instantaneously, allowing systems to adjust and respond dynamically. This real-time adaptability proves invaluable in scenarios such as smart cities, where the optimization of traffic flow, energy consumption, and public safety relies on immediate insights derived from continuously changing data.
- Improved Reliability and Redundancy: In scenarios where connectivity to central servers may be intermittent or disrupted, edge devices can continue to operate autonomously. This ensures that critical functions persist even in challenging network conditions, providing a level of robustness essential for applications with high-reliability requirements, such as industrial automation or healthcare monitoring systems.
Challenges and Considerations
Navigating the convergence of AI, edge computing, and data-in-motion brings challenges and considerations that demand careful attention. Here are key points to bear in mind:
- Bandwidth Limitations and Network Constraints: Efficient data compression strategies are crucial to mitigate strain on available bandwidth. Optimization techniques must be employed to ensure smooth operation and prevent network congestion.
- Security Considerations for Edge Devices: Robust security protocols and encryption mechanisms are essential to safeguard sensitive data. Regular updates are crucial to maintaining the integrity of converged systems, especially in diverse environments.
- Balancing Local Processing with Cloud Integration: Achieving an optimal balance requires thoughtful architecture design. Consideration of factors such as application requirements, data sensitivity, and overall system performance is paramount.
In the next section, we will explore how these benefits manifest in real-world applications across industries, showcasing the transformative impact of the synergy between AI, edge computing, and data-in-motion.
Applications Across Industries
These are some of the applications to keep an eye on as technology progresses.
Smart Cities: Real-time Optimization of Urban Services
AI, edge computing, and data-in-motion is revolutionizing urban living. Real-time data processing at the edge enables cities to optimize services dynamically. From traffic management that adapts to changing patterns in real-time to energy-efficient lighting systems responding to environmental conditions, smart cities leverage this convergence to enhance the quality of life for residents.
Industrial IoT (IIoT): Predictive Maintenance and Production Optimization
In the industrial landscape, the convergence is a game-changer for the Industrial Internet of Things (IIoT). Predictive maintenance, powered by AI algorithms at the edge, allows industries to anticipate equipment failures before they occur, minimizing downtime. The real-time analysis of production data optimizes manufacturing processes, enhancing efficiency and reducing operational costs. The result is a more resilient and responsive industrial ecosystem.
Autonomous Vehicles: Real-time Decision-making for Enhanced Safety
Autonomous vehicles rely on split-second decision-making, and the convergence of AI, edge computing, and data-in-motion is the linchpin of their success. Processing sensor data locally allows vehicles to make real-time decisions, ensuring enhanced safety on the road. From navigating complex traffic scenarios to adapting to sudden changes in road conditions, this convergence is at the heart of the evolution towards safer and more reliable autonomous transportation.
Future Trends and Innovations
Looking ahead, the convergence landscape is poised for exciting advancements. Here are key trends and innovations shaping the future.
Emerging Technologies Shaping the Future of AI, Edge, and Data-in-Motion
The future promises even more advanced technologies shaping the convergence landscape. Edge AI, federated learning, and edge-native applications are emerging as key trends. These technologies aim to push intelligence closer to the data source. This will reduce reliance on centralized cloud infrastructure and further enhancing the capabilities of edge devices.
Potential Advancements in Hardware and Software Solutions
Advancements in hardware and software are pivotal to the continued evolution of the convergence. Improved edge computing hardware, such as specialized AI chips and edge processors, will empower devices to handle increasingly complex computations. Similarly, software innovations, including more sophisticated AI algorithms and decentralized processing frameworks, will drive efficiency and scalability.
Predictions for the Continued Impact on Technology Landscapes
As the convergence matures, it is expected to profoundly impact technology landscapes across diverse sectors. The seamless integration of AI, edge computing, and data-in-motion from healthcare to agriculture will foster innovation, create new business models, and drive efficiency. The continued democratization of these technologies will empower organizations of all sizes to harness the benefits, leading to a more interconnected and intelligent future.
Recapping Transformative Synergy
The convergence of AI, edge computing, and data-in-motion marks a transformative paradigm shift, unlocking unprecedented possibilities. Businesses and industries are urged to embrace this synergy for enhanced efficiency and innovation. As we progress, the potential for continued growth and advancement is vast. It promises a future where the seamless integration of intelligence and real-time processing reshapes the technological landscape. Embrace the convergence and usher in a new era of interconnected, intelligent solutions.
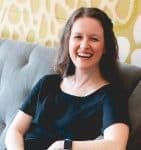
Elizabeth Wallace is a Nashville-based freelance writer with a soft spot for data science and AI and a background in linguistics. She spent 13 years teaching language in higher ed and now helps startups and other organizations explain – clearly – what it is they do.