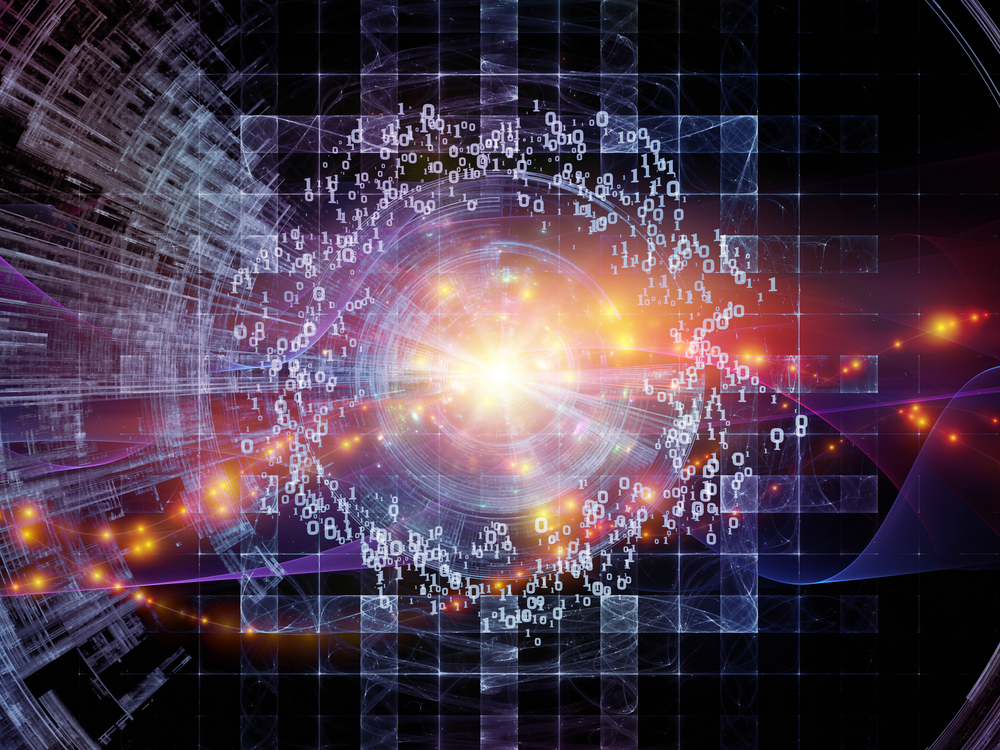
The days of manual data pipeline creation are fading fast if not already long gone. The modern data stack is too complex, and the volume of pipelines needed in most businesses makes data pipeline automation a must. Fortunately, many new data pipeline technologies are coming to market that can help. As this is happening, some are wondering how this will impact the role of the data scientist.
To put things into perspective, let’s take a step back on both fronts (the need to automate data pipelines and the changing role of the data scientist).
Data pipeline technologies abound
Organizations find they must quickly build data pipelines to meet the demand of the business. The data is typically needed for reporting, analytics, or other applications. Regardless of the project, the data scientists and line of business users that need the data do not have the expertise to connect to a source, put the data in an appropriate format, convert it (if needed), transport it, and securely get it into the right hands at the right time.
In the days when there were only a handful of requests for new pipelines a month, IT and data engineers could do the work in a reasonable time. But most organizations now routinely need tens, hundreds, thousands, or more pipelines built a year.
The obvious issue is the time it would take such teams to build the pipelines. But there are added problems. The modern data stack is increasingly more complex. It takes many more tools and people with special skills to put pipelines in place. That is happening at a time when organizations are having trouble finding qualified people to do these jobs.
Given these issues, many organizations are looking for technical solutions to help automate data pipeline creation. Here are some of the things they are evaluating or using:
Low-code/no-code solutions: Such solutions let non-technical people build pipelines using a visual programming environment. They can drag-and-drop elements that need to be connected or insert actions that need to be taken (e.g., a data transformation).
Generative AI: ChatGPT created an explosion in interest in generative AI. There are several ways it can be used to help build data pipelines. Some companies are trying to enhance their data stack tools. Many of these tools are often complex and difficult to use. Some vendors are now adding a generative AI front-end that makes it easier to access different features of the tool.
Other vendors and some data scientists, and data engineers are using generative AI to speed up manual programming. They use the technology as a way to verbally describe the code that is needed for pipeline creation. The app then generates the appropriate code.
A cloud assist: Many organizations are constantly moving data into and out of cloud databases, data lakes, data warehouses, and more. Or they are making use of cloud compute power and algorithms to do their analytics or train and run AI and machine learning routines. To help, all of the major cloud providers now offer connectors, APIs, and tools that support and manage the needed data flow.
See also: The Evolving Landscape of Data Pipeline Technologies
Where does the data scientist fit in?
It is easy to say the role of the data scientist is changing, especially given all the new technologies that are now available. But some core things never change. The introduction of electronic calculators made it much easier to do basics like long division (without a slide rule), percentages, and simple analytics. But those using the technology still had to understand how to set up a problem. The same holds true today, even with all of the new technology that assists data scientists and others when they need a data pipeline.
About ten years ago, this point was made in a landmark Harvard Business Review (HBR) article titled: Data Scientist: The Sexiest Job of the 21st Century. The authors, Thomas H. Davenport, the President’s Distinguished Professor of Information Technology and Management at Babson College, a visiting scholar at the MIT Initiative on the Digital Economy, and a senior adviser to Deloitte’s AI practice; and DJ Patil, the first U.S. Chief Data Scientist, and currently a board member for Devoted Health, a senior fellow at the Belfer Center at the Harvard Kennedy School, and an adviser to Venrock Partners; noted data scientists are “the ones who can coax treasure out of messy, unstructured data.”
They also noted at the time that “the dominant trait among data scientists is an intense curiosity—a desire to go beneath the surface of a problem, find the questions at its heart, and distill them into a very clear set of hypotheses that can be tested. This often entails the associative thinking that characterizes the most creative scientists in any field.”
To emphasize this point, they highlighted an example of a data scientist studying a fraud problem who realized that it was analogous to a type of DNA-sequencing problem. “By bringing together those disparate worlds, he and his team were able to craft a solution that dramatically reduced fraud losses,” they said.
What’s changed since then? The authors gave an update in a new HBR article: Is Data Scientist Still the Sexiest Job of the 21st Century?
They noted that in the original article, “many data scientists spent much of their time cleaning and wrangling data, and that is still the case despite advances in using AI itself for data management improvements.”
And the job has changed in other ways over the decade since the first article came out. The job has become better institutionalized, its scope has been redefined, the technology it relies on has made huge strides, and the importance of non-technical expertise, such as ethics and change management, has grown.
That latter point is increasingly important. The authors noted that the job of data scientists will only continue to grow in importance in the business landscape.
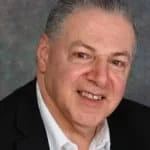
Salvatore Salamone is a physicist by training who has been writing about science and information technology for more than 30 years. During that time, he has been a senior or executive editor at many industry-leading publications including High Technology, Network World, Byte Magazine, Data Communications, LAN Times, InternetWeek, Bio-IT World, and Lightwave, The Journal of Fiber Optics. He also is the author of three business technology books.