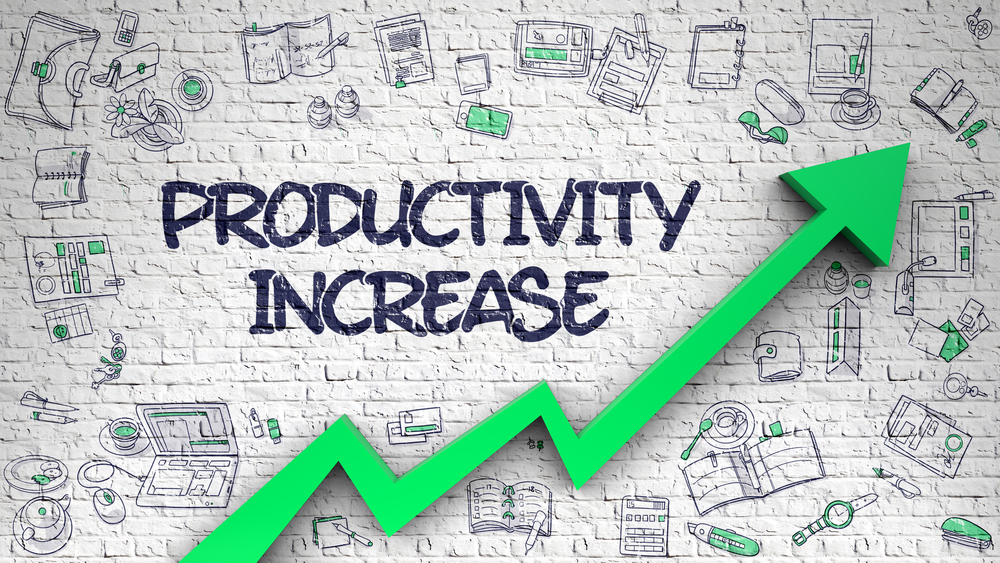
It’s an old story. New technology is supposed to make life more efficient, but it simply adds more to an already full plate. As the data landscape evolves, many organizations find their data teams struggling to keep up with the challenges of growing data volumes and implementing new data tools. To navigate this shifting terrain successfully, it’s essential to follow a clear roadmap that consists of three crucial steps: understanding the challenges, addressing pitfalls to align with business objectives, and implementing effective strategies. When data teams harness generative AI, it can make the goal of better productivity even more reachable.
Step 1: Understand the complexities and challenges of the current data landscape
In today’s data landscape, data teams encounter an array of challenges:
- Data Variety and Volume: The data explosion has brought data in various formats, including structured, semi-structured, and unstructured data. For instance, a retail company may need to handle sales transaction data, customer reviews, and social media sentiment analysis, all of which come in different formats and sizes.
- Data Silos and Integration: Data often resides in isolated silos across an organization. Consider a healthcare system where patient records are stored separately from financial data, making it challenging to gain a holistic view of patient care and operational efficiency.
- Data Quality and Governance: Maintaining data quality is an ongoing battle. In the financial sector, a minor error in financial data can have significant consequences, from inaccurate financial reporting to regulatory compliance issues.
- Skill Shortages: The demand for data professionals continues to outpace supply. For example, the demand for skilled data scientists has grown substantially across industries, from e-commerce to healthcare, making talent acquisition highly competitive.
- Technological Complexity: The rapid evolution of data technologies, such as cloud platforms and machine learning tools, requires data teams to constantly update their skill sets.
See also: Transforming Data Engineering with Generative AI
Integrating GenAI: Enhancing data understanding
Generative AI can assist data teams in understanding complex data patterns and anomalies. It aids in data exploration by processing massive datasets and generating textual summaries. For example, in financial services, generative AI can quickly analyze financial reports and produce concise summaries, helping data teams identify trends and outliers more efficiently.
Step 2: Address the overlooked pitfall — lack of alignment with business objectives
Aligning data efforts with business objectives can significantly impact productivity. Here are some examples:
- Sales Growth: A retail company’s data team focuses on customer segmentation and personalized marketing strategies to boost sales revenue, aligning its efforts with the overarching business goal of revenue growth.
- Cost Reduction: In the manufacturing sector, a data team may analyze operational data to identify inefficiencies, thereby supporting the organization’s objective of reducing production costs.
- Customer Satisfaction: A telecommunications company aligns its data initiatives to improve customer satisfaction by analyzing customer feedback and network performance data to enhance service quality.
- Compliance: In the financial industry, data teams ensure that their work aligns with regulatory compliance objectives, such as anti-money laundering (AML) regulations or Know Your Customer (KYC) requirements.
Integrating GenAI: enhanced communication and alignment
Generative AI can bridge the communication gap between data teams and non-technical stakeholders. It can generate clear, plain-language explanations of data findings, making it easier for business leaders to understand and align with data-driven recommendations. For instance, it can generate non-specialist’s terms explanations of how a predictive model works, helping business leaders appreciate its potential impact on sales growth.
Step 3: Implement strategies for enhanced data team productivity
To empower data teams, organizations can implement various strategies, each tailored to specific challenges:
- Clear Goal Definition: To align data projects with business objectives, organizations can set clear and specific goals. For example, a healthcare provider might set a goal of reducing patient readmission rates by a specific percentage using predictive analytics.
- Alignment with Business Objectives: Regular communication with business leaders and stakeholders helps ensure that data initiatives remain aligned with evolving business needs. A real estate company aligns its data strategy with its objective to expand into new markets by analyzing market data and trends.
- Continuous Evaluation: Organizations should establish metrics to track progress continually. In the e-commerce sector, a company monitors conversion and shopping cart abandonment rates to measure the effectiveness of its data-driven recommendations.
- Team Education: Ongoing training and development programs ensure data team members have the latest skills and knowledge. For instance, a data analytics team participates in regular workshops to stay updated on data visualization techniques.
- Feedback Loop: Establishing a feedback loop with business stakeholders is essential. In the energy sector, a utility company collects customer feedback to improve data-driven energy consumption recommendations.
Leveraging GenAI as a strategic tool
Thoughtful GenAI integrations can enhance data team productivity. Here are several ways companies can leverage it now.
Automating repetitive tasks
One of the significant advantages of generative AI is its ability to automate repetitive tasks. Data teams often spend significant time on data cleansing and report generation. Generative AI can automate these tasks, allowing data professionals to focus on higher-value activities. For instance, it can automatically generate regular data quality reports, highlighting areas that need attention.
Efficient data model training and deployment
Generative AI can streamline the process of training and deploying machine learning models. It can assist data teams in generating model code, hyperparameter tuning scripts, and deployment pipelines. This accelerates developing and deploying data-driven solutions, allowing organizations to reap the benefits of advanced analytics faster.
Enhanced data documentation and cataloging
Managing data documentation and cataloging can be time-consuming. Generative AI can automate the creation of data dictionaries, metadata, and data lineage documentation. It can also generate natural language descriptions for datasets, making it easier for data teams to understand and utilize available data assets efficiently.
The synergy of generative AI and data teams
By leveraging generative AI, data teams can streamline tasks, improve communication, and accelerate insights generation. However, using generative AI thoughtfully is essential, ensuring that it complements human expertise and aligns with the organization’s goals. As the data landscape continues to evolve, the synergy between data teams and generative AI represents a powerful partnership. Together, they can unlock new dimensions of productivity and innovation, enabling organizations to thrive in an increasingly data-driven world.
This journey is not just about technical proficiency; it’s about creating a symbiotic relationship between data initiatives, business goals, and new technologies like generative AI that drive the organization forward. With a keen focus on these strategies and alignment with broader objectives, organizations can harness the power of their data teams to extract valuable insights and drive innovation in a data-rich world.
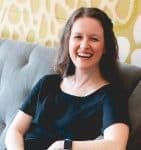
Elizabeth Wallace is a Nashville-based freelance writer with a soft spot for data science and AI and a background in linguistics. She spent 13 years teaching language in higher ed and now helps startups and other organizations explain – clearly – what it is they do.