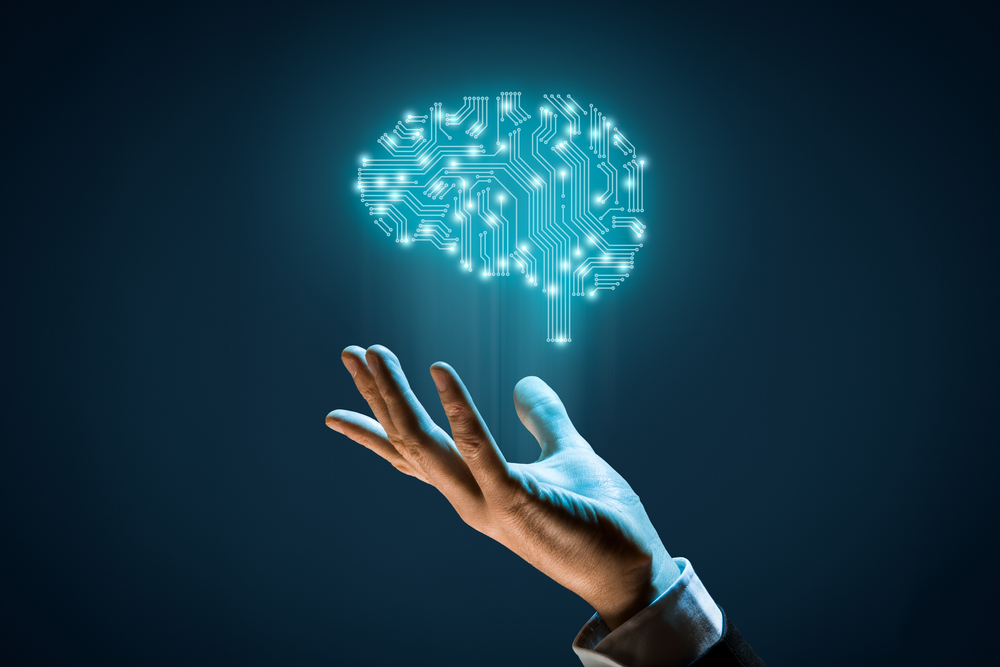
Machine learning is already being applied in many industries, and 2022 was a breakthrough year in terms of consumer adoption with the launch of DALL-E and ChatGPT. The next few years will see broader adoption of the technology, with an expected market size of over $100 billion by 2030.
At the recent AWS Re:Invent conference, Bratin Saha, vice president and general manager of AI and ML at AWS, outlined six key machine learning adoption and innovation trends that are pushing forward the technology in 2022 and beyond.
See Also: Retail is the Ultimate Testing Ground for AI/ML
- Model Sophistication
The number of parameters in machine learning models has grown exponentially in the past few years, from a few hundred million in 2019 to over 300 billion in some of the most sophisticated models.
“In just three years, the sophistication of machine learning models has increased by 1,600 times,” said Saha. Foundation models such as these open the doors to a wide variety of applications, and can be repurposed with a few changes, as we saw with DALL-E, a reworked version of GPT3.
See also: Extending Data Warehousing to the Cloud
- Data Volumes and Variety
Parameters and data growth go hand in hand, with increased volumes and variety of data being used to train ML models. Organizations involved in building models are utilizing text, video, and audio data, and looking further afield to acquire rich datasets.
New applications are also enabling model makers to process unstructured data and make it practical for machine learning models, vastly enhancing the value of crawling the web for any sort of data.
- Machine Learning Industrialization
Industrialization of machine learning means that standards and infrastructure are being more readily applied throughout the industry, leading to faster application development.
“Even within Amazon we are using SageMaker for industrializing and machine learning development,” said Saha. “For example, the most complex Alexa speech models are now being trained on SageMaker.”
- Specific Use Cases
Machine learning is now being applied for specific use cases, rather than organizations simply looking for ways ML will impact the entire company. More customers are building specific applications which utilize AWS’ voice transcription, translation, text-to-speech, and anomaly detection.
- Responsible AI
More vendors and developers are practicing “responsible AI”, which aims to reduce bias in a system and operate equally regardless of attributes, such as race, gender, or religion. Developers are also improving the amount of explainable items inside their system, allowing non-technical users to understand how the model operates and why it has made a decision.
“With that growth in AI and ML comes the realization that we must use it responsibly,” said Saha.
- Democratization
The further democratization of machine learning by making tools and skills accessible to the largest amount of people will also help ML adoption and help it evolve. Low-code initiatives and standardization of tools and infrastructure should provide developers without ML experience with the ability to step into AI and ML fields, which are expected to be the most hamstrung by lack of data science talent.
“AWS is also investing in training the next set of machine learning developers,” Saha said. “Amazon has committed that by 2025 we will help more than 29 million people improve their tech skills through free cloud computing skills training.”
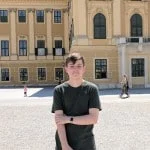