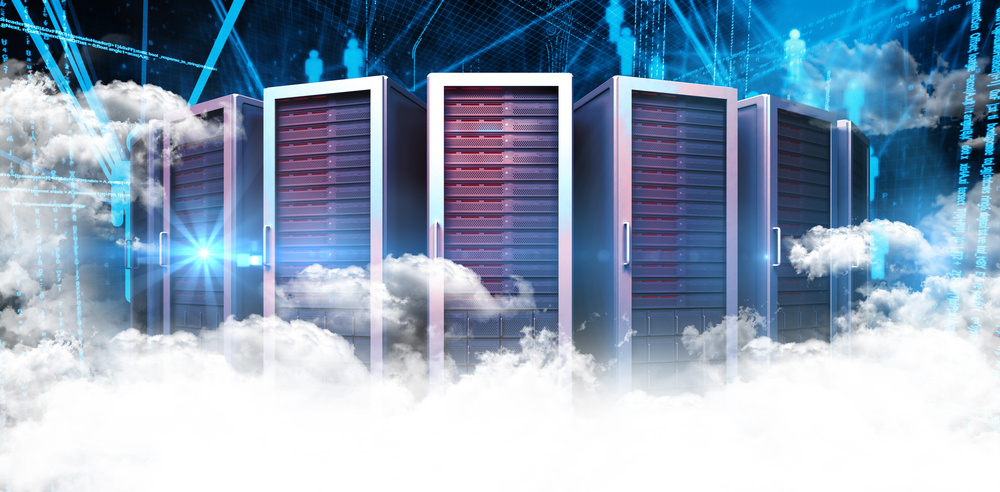
From large, on-premises Hadoop farms to streaming ingestion into everything, everywhere, SteamSets’ Mike Pickett has seen it the gamut. His take: No matter the technical preferences or technology hype, the architecture must meet business needs above all.
Cloud migration is proceeding at a rapid pace, but for many organizations, there are unanswered questions about what to move where, where should what kind of processing occur, and how one decides. Some of the factors to consider are the size of the data, the importance of broad access, the speed of results, data gravity, security, regulations, and the cost–of migration, maintenance, and the eventual ROI.
Mike Pickett, the Vice President of Growth for StreamSets (now part of Software AG), sat down to talk with Cloud Data Insights (CDI) at the 2023 Gartner Data & Analytics Summit about how organizations leverage or ignore their trove of historical enterprise data and how they got to this point. (See his bio below.) Here are excerpts from our conversation with his thoughts on various topics.
Data warehousing disruption
StreamSets’ heritage goes back to first-generation Hadoop and the on-premises world. StreamSets disrupted what ingestion was by allowing you to bring in everything, even if the format changes in the source, so that you don’t have data engineers spending time reworking mappings. Once the data is in the destination, you can do whatever you want with it. In the world of legacy data warehousing, you cleaned everything up because the cost of having data in the data warehouse was upstream. In the big data world, it gets less expensive. It’s amazing what you can solve with commodity hardware. But it was still an incredibly complex product stack. And now we’re well into this next phase of the cloud.
Building a good analytics project
One of the trends that I see, and Gartner sees, is that process is not the way to define good analytics and projects–it’s the ability to always bring more and new data to the process. Good analytics are a result of an iterative and ongoing refinement–it’s not the big ban. For example, for manufacturers to improve just 1% might not seem like much, but if you’re doing fast loops of improvement, ten loops give you a 10% improvement. Don’t try to moonshot. We see that a lot of buyers have their processes down; they know how to run a good analytics project and spot business outcomes.
The cloud definitely facilitated that because now business analysts have proven that they can get up and going with cloud technology. There’s no need to go to Central IT and ask, “Can you stand up this?” and “Can I get this data in”? They can do it on their own. They can do it with Snowflake, Amazon, DataBricks, and Google Big Query, to name a few.
The critical role of Central IT
A theme I’ve frequently seen is that sales and marketing teams drove a huge amount of this early cloud adoption. They’ve got information from this morning’s ad spend and need to change something right away, like this afternoon. Central IT would have you submit a trouble ticket, and you might get your request three weeks later. A friend who runs a medical device company with a multimillion-dollar quarterly spend needs to make changes twice a day. By using a product called Stitch Data, she sees the whole data model in 15 minutes. Fivetran provides the same capability.
Now that sales and marketing proved what they could do with their SaaS application data, they’re coming for the enterprise data and Central IT. Unfortunately, it’s not that easy. They have the tools that let them use data on their own, but IT is the group that can ensure security measures throughout the infrastructure: firewalls, message queues, decades of legacy infrastructure, and custom applications, to name a few.
Conversations around cloud migration are changing
Different trends are emerging here at the Gartner Data & Analytics Summit. I’ve heard a lot of people saying that they’re using one set of tools to get data into one cloud and another set for a different cloud. Or a different tool to get the data to where they can use the cloud migration tool. The problem we’re having is training people on different tools and doing a lot of troubleshooting. When you’re working with multiple sources and targets, it’s hard to find the problem, work through formatting issues and lineage questions, and finally isolate the cause. People are looking for a single tool that lets them deal with a variety of sources and targets.
Accessing the wealth of enterprise data
Along with decades of infrastructure, you also have decades of data–decades of information. Enterprise is so deep, and it’s complex, it’s diverse, it’s secure. Take the iceberg analogy. The application data is the tip of the iceberg–it’s what business groups have cared about. The bulk of an organization’s data wealth is below the water line.
The challenge we see is, how do you get to this data for the cloud? We see a lot of traditional approaches, such as hand-coding or using tools that weren’t designed for the cloud. When these tools are used for cloud migration, they can break, and then you have data engineers spending time fixing systems.
See also: Beyond Mainframe Data Access: the Need for Smart Data Pipelines
Unfortunately, one approach we see customers take is to simply ignore their legacy enterprise data. A saying I like is, “One man’s garbage is another man’s gold.” A word of caution here–yes, there’s gold in the enterprise data, but the requester has to prove the need. Sometimes, tactical prioritization happens, and the job that can be done the fastest gets picked up first, and the one that will have more impact on the business waits in the queue. While the analysts know the potential impact, Central IT won’t.
The tension between agile reporting and regulatory reporting
Both kinds of reporting typically look at the same enterprise data repository (data warehouse, data lakehouse, which results in contention and prioritization issues.) Agile reporting is a more flexible kind of enterprise reporting. But regulatory reporting is not at all flexible. You’re reporting ESG data now in addition to all manner of compliance data. With deglobalization, you have to report different data sets in different formats for specific countries and regions. ESG reporting requirements are converging, but data sovereignty and privacy laws are fracturing.
See also: ESG Leadership needs to come from the leaders
The ideal is to automate all that reporting, but you have to adjust constantly for changes to reporting standards. The leading organizations are applying advanced analytics to their regulatory reporting efforts to find areas of risk which is something the data scientists specialize in.
Regulatory reporting brings up some new requirements that some platforms are better suited to handle than others. Snowflake and DataBricks are the ones we hear most about. Both have advanced analytics tools (Snowpark, e.g.). What we’re seeing is some of the advanced analytics applied to other use cases like spotting trends, churn, and identity fraud are being applied to regulator reporting.
And all of this is being fueled by the cloud. I predict you’re just going to go deeper and deeper to get your enterprise data.
The future of enterprise data is the cloud and the mainframe
What you get at the end of the day is one pipeline from the source to the landing zone and the whole data lake concept of bronze, silver gold, but it landed where anyone can choose to access the data from that source. They don’t have to go back to the original, original such as a mainframe or a Teradata system. The enterprise data is there, it’s holistic, and it’s a good pipeline. You can then have multiple people leveraging it for their own use case.
See also: Bringing Data Pipelines and Strategy into Focus
The mainframe use case is usually very strategic to the business. It’s transactional, and it can have deep historic data. The mainframe is largely left in place where it serves the need for transactional integrity and data processing scale. The same can go for other operational systems. For instance, we see a lot of transactional systems, Oracle, SQL Server, and Postgres, that companies have built applications over. And they wanted to move those into the cloud for use cases such as operational reporting or the opposite–deep discovery work. The cloud gives them the ability to serve both constituents. I’ve learned of one company that spent $250 million to get off a mainframe, only to find out they could not do it.
A lot of CEOs are telling me that if there’s one thing they could do, they would get their kids to take COBOL for life-long guaranteed employment.
Let me be clear, though–it’s not just about the mainframe–we care about all enterprise data, whether it be on a mainframe, in one of many clouds, or on an on-premise open system. Organizations have an imperative to open the enterprise data treasure to other systems, and they must find a secure, cost-effective way to do that. This is not a case for cloud migration. Keep the legacy systems running AND work on their data in the cloud.
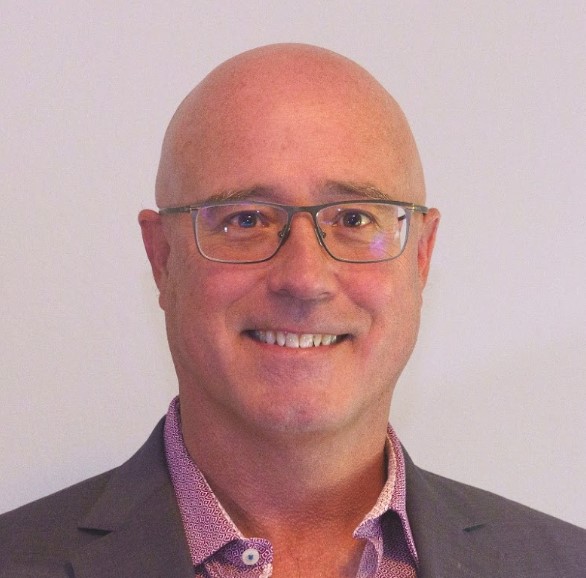
Bio: Mike Pickett is the Vice President of Growth at StreamSets. He is a veteran in the technology industry and a specialist in the data integration market. Mike has held Sr. Leadership roles at Tibco Software, Informatica, and Talend, spanning Alliances, Sales, Strategic Business Development, and Corporate Development. In his role as Vice President of Growth at StreamSets, Mike is responsible for overseeing the Developer self-service and Product Led Growth initiatives.
Elisabeth Strenger is a Senior Technology Writer at CDInsights.ai.