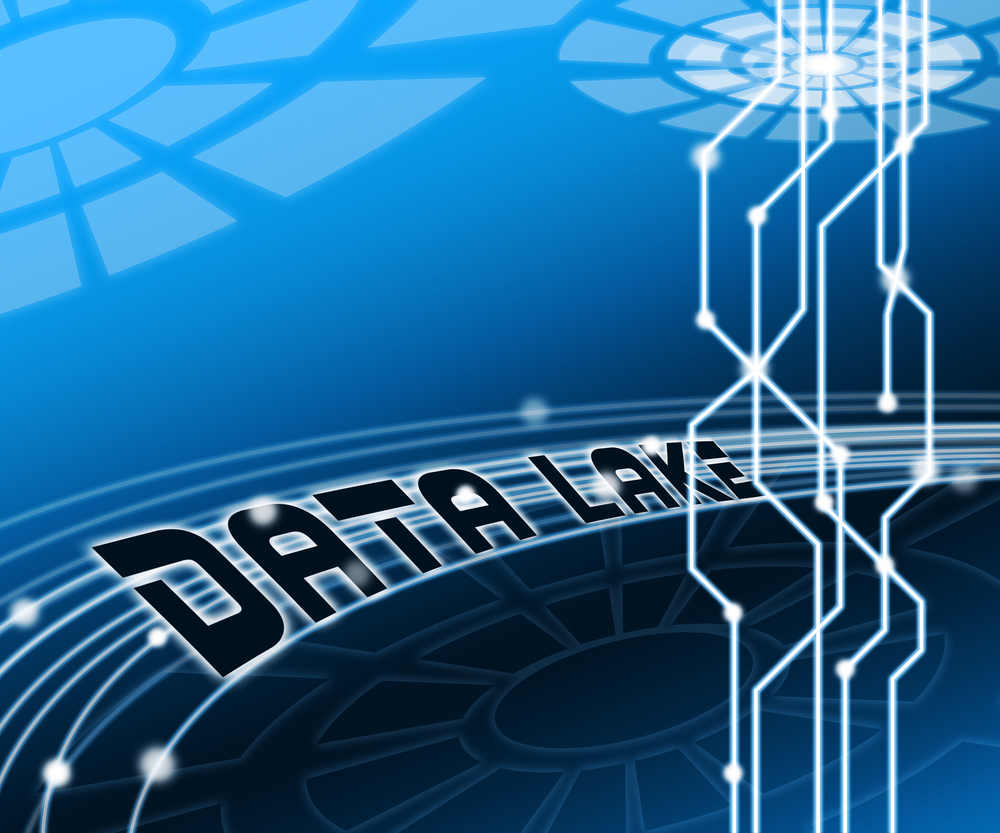
Data lakes have the ability to store a variety of data types and rapidly handle the huge volumes of data, which has led to their widespread adoption. Gartner defines a data lake as a collection of storage instances of various data assets that are stored in a near-exact, or even exact, copy of the source format of the originating data stores. So, data lakes hold enormous promise in supporting modern enterprise data architectures. Implementations continue to be successful in uniting enterprise data physically; however, they can fall short in delivering returns for business users. This is because the bulk of the data within the data lake is unconnected and stored in its native form, requiring businesses to spend considerable time and money to prepare it for analysis.
See also: What is a Data Lakehouse?
When used in conjunction with data lakes, data lakehouses, an approach that combines elements of the data warehouse with those of the data lake, help organizations co-locate data from across the organization using cost-effective approaches for storage. They also provide the opportunity to leverage the data at the computational layer to capitalize on the benefits of AI and reduce the need to maintain expensive and brittle ETL pipelines against traditional structured and costly on-prem data warehouses. However, while data lakes address the data access problem, they have yet to democratize access so that non-technical users can self-serve and collaborate to generate the rapid insights needed to keep pace with consumer preferences and changing business dynamics.
See also: The Role of Knowledge Graphs in Cloud Data Integration
In the past, organizations linked BI tools to their data lake, but this resulted in other issues, such as higher latency, reduced collaboration and reuse, and the inability to leverage data across domains to provide context. These storage solutions also hindered the ability to conduct self-service through data exploration in support of enriching analytics and inferring new insights.
To resolve those challenges, organizations are adopting modern data management approaches such as enterprise knowledge graphs to connect data across the enterprise and accelerate the value from their data lake investments. By connecting enterprise data with business semantics, knowledge graphs reduce the cost of data integration and help generate powerful insights into complex business challenges, all while enabling more agile data operations.
Read the rest of this article on RTInsights.
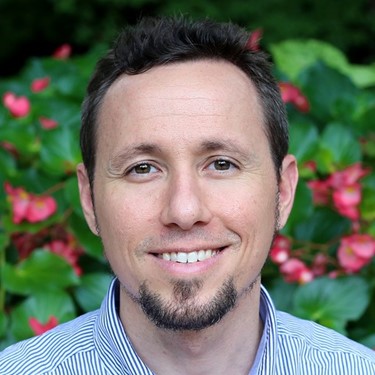
Brendan Newlon is a Solutions Architect at Stardog, the leading Enterprise Knowledge Graph (EKG) platform provider. For more information, visit www.stardog.com or follow them @StardogHQ.