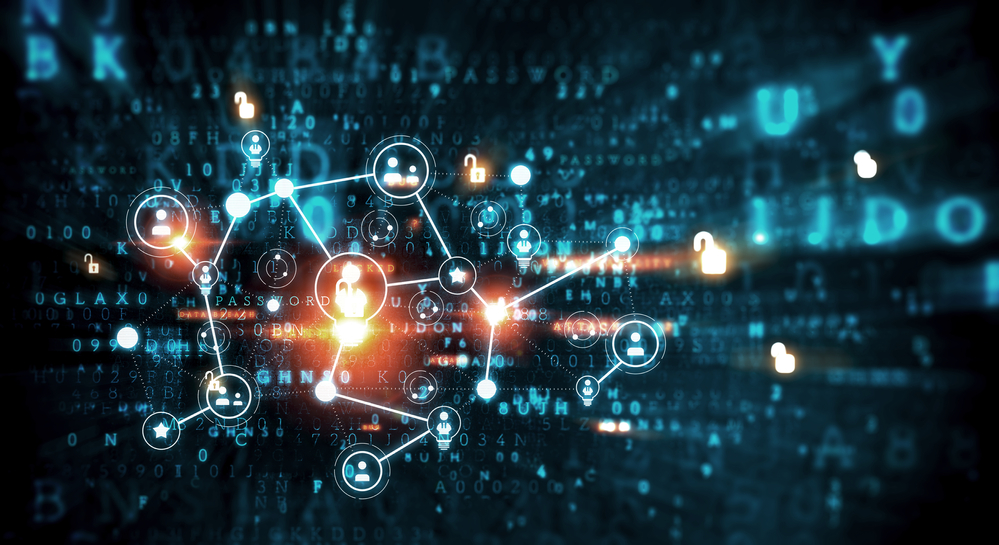
Multiple studies over the years have documented the rise in the use of additional data sources in decision-making processes. Tellingly, most companies are incorporating external data sources to complement their own resources.
About five years ago, one study found that about half of all companies used less than five internal and five external data sources for decision making. Fast forward a few years, and a Deloitte report found that the number of external data sources used by businesses had exploded. And yet another survey claimed that the mean number of data sources used by businesses was closer to 400, with some saying they used more than 1,000 sources.
Why the great shift? The move to more data sources is being aided by the use of cloud databases, standard APIs such as OpenAPI, and more. Additionally, the inclusion of additional and different data sources into business intelligence and analytics routines used for decision making can deliver significant improvements in the insights derived from data. For example, a McKinsey study noted the work of an insurer that transformed its core processes, including underwriting, by expanding its use of external data sources. The insurer went from a small number of sources to more than 40 in a two-year period. The result: the company was able to increase the predictive power of its core models by more than 20 percent.
The insights gained from the additional sources provided more information about customers. That allowed the insurer to eliminate many questions it would previously ask on customer applications. Having fewer questions, the complexity of the application process was reduced, enabling more customers to successfully complete the process.
See also: Challenges Data Pipeline Engineers Need to Overcome
It’s not just the data, complexity abounds in other ways
Having numerous data sources introduces countless issues. Much of the data is housed in tools, many of which are unconnected and use proprietary technologies. One study tried to put the scope of the issue into perspective. It found that, on average, companies have about 20 different technology applications leveraging their data sources.
That may not be the case for all businesses, but the issue of tool sprawl with respect to accessing, transforming, transporting, and managing data is critical in modern businesses today. There is much talk about how complex the modern data stack has become. And that, in turn, is impeding businesses from reaping the potential benefits more data can theoretically provide.
Let’s look at the issues.
Key elements that make up a modern data stack typically include tools for data ingestion, transformation, orchestration, observability, and data delivery (or what is known as reverse ETL). Each tool overcame a different pain point data engineers dealt with when trying to build data pipelines and provide data consumers with access to their needed data.
A business would often pick tools in each category that were considered the best in class at a given time. Data engineers would then have to integrate these tools with the existing data infrastructure. That all sounds straightforward. But problems start from the get-go. The tools themselves might not easily integrate. Many will evolve over time, with some becoming unavailable and unsupported.
Then there is the issue of scaling. As noted, businesses are adding new data sources all the time. The tools in place to manage and work with data sources on one day may not be of use when new data sources are introduced. So, the company must add more tools.
You can see where this is going. Scalability quickly becomes an issue. Large amounts of time are consumed on managing and integrating tools. That leaves less time to work with the data.
Further complicating matters is the issue of skills. Staff well-versed in data center-centric data stack tools may be out of their element when the cloud, data lakes, and other modern technology become part of the data stack.
See also: 3 Ways to Improve Data Team Productivity in the Age of Complexity
Enter the post-modern data stack and data pipeline automation
To overcome the issues as more and more data sources are used, businesses are looking to what some call the post-modern data stack and data pipeline automation.
At a very high level, a post-modern data stack seeks to abstract the nuances of the individual tools. Instead, businesses rely on a system that integrates all of the needed functions and provides access to capabilities via a single interface.
With respect to data pipeline automation, the goal is to evolve from using numerous interfaces for different tasks to a single, consolidated platform. This means if a pipeline needs to be built or fixed, it can all be managed within one unified interface.
Such approaches will help businesses make use of any additional data sources they feel would improve their operations, analyses, and bottom line.
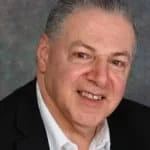
Salvatore Salamone is a physicist by training who has been writing about science and information technology for more than 30 years. During that time, he has been a senior or executive editor at many industry-leading publications including High Technology, Network World, Byte Magazine, Data Communications, LAN Times, InternetWeek, Bio-IT World, and Lightwave, The Journal of Fiber Optics. He also is the author of three business technology books.