
Volume, variety, and velocity of data have exponentially increased, and now every company is potentially a data company. Organizations strive to leverage data for competitive advantage, taking advantage of an abundance of tools and well-trained talent – whether from in-house teams or SaaS support – designed to manage this volume and streamline analysis. Why then are data teams facing unprecedented challenges in managing and extracting value with this abundance of information and solutions? The answer is the complexity of today’s data landscape, something that a combination of people, processes, and modern technology can address.
Understanding productivity challenges
Data teams face a lot in pursuit of managing data.
- It’s not just volume; it’s variety: Teams must efficiently collect, organize, and integrate data from diverse systems and formats, creating a time-consuming and resource-intensive ecosystem.
- Lack of streamlined data access and integration: Teams may struggle with accessing and integrating data from these disparate systems. Siloed data and disparate tools hinder the data flow/workflow and lead to delays.
- Conventional data analysis lags: Slow query performance and data transformation processes delay the generation of insights and value. Even worse, continued (unnecessary) manual processes like data cleansing hamper productivity.
- Continued governance and privacy concerns: Data teams must navigate increasingly more complex regulatory requirements and protect sensitive information. This adds extra pressure on teams before they can ever get insights.
- Talent shortages and employee attrition: Even with more people training for data science-related jobs, technology changes quickly, and talent moves on just as quickly. One study suggested talent tends to stay in a single position for less than two years, which makes consistency a challenge.
How can organizations support data teams? It is possible to overcome productivity challenges with the right support.
See also: What’s Changing Faster? Data Pipeline Tech or the Role of the Data Scientist?
Enhancing data productivity despite complexity
Organizations can mitigate the effects of complexity by leveraging a combination of strategic tools, new technologies, and collaboration.
Implement advanced data management and integration tools
Just because tools aren’t magic bullets, it doesn’t mean tools aren’t a critical part of the data management plan of attack. They can help smooth out changeover as data team members leave and others come on board and encourage more participation from other stakeholders in the organization.
- Explore data integration platforms: Modern data integration platforms provide a comprehensive solution for data access, transformation, and integration challenges. The key is getting all data sources and formats into some kind of universal view.
- Leverage better data virtualization: Instead of creating copies of data (multiplying weaknesses and complexity), virtualization allows teams to access and integrate data from multiple sources without moving or duplicating it.
- Adopting the cloud: Strategically migrating data infrastructure to the cloud reduces maintenance efforts, provides on-demand resources, and facilitates collaboration. Although there are many considerations to cloud migration, it remains a critical part of a data strategy.
Automate, automate, automate with AI
AI isn’t going to replace humans, but it will help humans do better work.
- Utilize machine learning for data preprocessing: Data cleaning, normalization, and feature engineering require tons of manual effort, but AI can take care of these tasks while humans focus more on analysis and insight generation.
- Automate repetitive tasks and workflows: The more time data teams have to work on higher-value activities, the better chance they’ll extract real value from data.
- Apply AI-driven analytics and insight generation: Teams can even go one step further with AI to help identify patterns from large datasets and enable data-driven decisions. Automating more complex tasks where available accelerates insight generation and improves productivity.
Bring in people: Establishing a collaborative data culture
Collaborative data culture is a strong component of a data strategy. Fostering cross-functional collaboration and communication between data teams, business stakeholders, and IT departments fosters shared responsibility and understanding. Integrating collaboration tools and practices enable efficient communication, knowledge sharing, and alignment boosts productivity.
This doesn’t mean bringing in every stakeholder for each micro-segment of the pipeline and strategy prep. It does mean a careful alignment between stakeholders to ensure business alignment and clear goals.
Data teams can benefit from the knowledge-sharing inherent in collaboration. Stronger knowledge bases empower teams to tackle complex challenges more effectively. Organizations can encourage this through investments in knowledge-sharing platforms and regular training sessions to re/upskill data team members. In fact, a complementary culture of continuous learning—fostered through workshops, conferences, and other professional development opportunities—helps both data teams and potential citizen data scientists expand their role in the organization’s data strategy.
Agile methodologies foster collaboration in data projects because of the iterative nature of the DevOps process. Teams improve through incremental development, providing continuous feedback and faster delivery for a more dynamic approach to data strategy.
Today’s data teams need new solutions
Modern data teams face so many challenges that hinder productivity and prevent companies from using data to produce value. The overwhelming volume and variety of data, fragmented access and data silos, and inefficient manual processes pose significant hurdles for data teams. However, there are ways organizations can support data teams and unlock the full potential of their data.
As the data landscape evolves, it’s essential to find solutions that work with the complexity of the modern data space and take proactive steps to overcome these obstacles. What worked in the past won’t work now. Assessing current practices is a must, along with identifying areas where improvements could move the business needle. A holistic approach encompassing technology solutions, processes, and people will streamline data workflows and overcome many of the challenges facing organizations today.
There’s nothing to be afraid of. The future still holds immense potential for data team productivity despite challenges, and advancements in technology and tools will help data teams thrive. Once data teams embrace the need to adapt, they’ll play a vital role in harnessing the power of data for decision-making. The future belongs to those who can effectively navigate the evolving data landscape.
Additional Reading and Resources:
The Evolving Landscape of Data Pipeline Technologies
The Hidden Challenges of the Modern Data Stack
What is Data Pipeline Automation? (on Ascend.io)
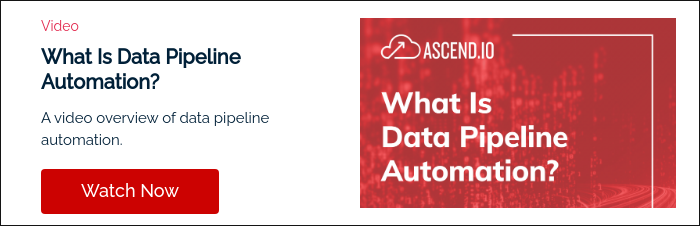
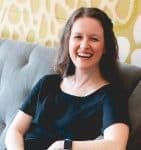
Elizabeth Wallace is a Nashville-based freelance writer with a soft spot for data science and AI and a background in linguistics. She spent 13 years teaching language in higher ed and now helps startups and other organizations explain – clearly – what it is they do.