Center for Data Pipeline Automation
As businesses become data-driven and rely more heavily on analytics to operate, getting high-quality, trusted data to the right data user at the right time is essential. Read more
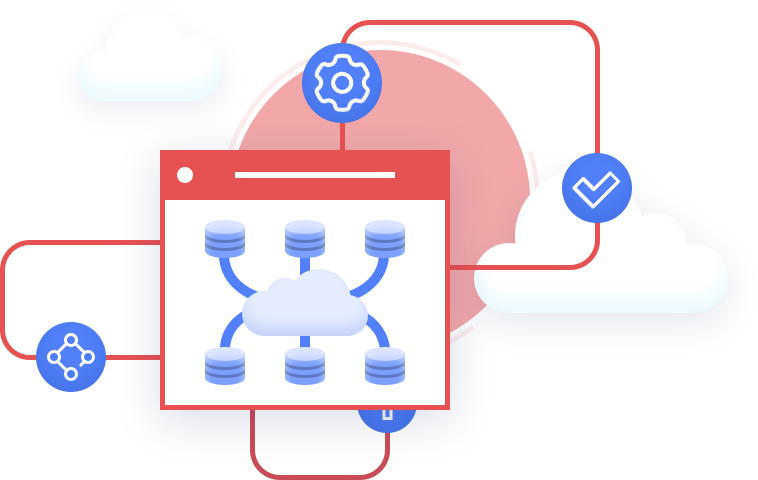
A Roadmap to Boost Data Team Productivity in the Era of Generative AI
By leveraging generative AI, data teams can streamline tasks, improve communication, and accelerate insights generation.
What Is Data Pipeline Automation?
Theoretically, data and analytics should be the backbones of decision-making in business. But for most companies, that’s not the reality.
Resources
Unlocking Autonomous Data Pipelines with Generative AI
Data engineering is changing. Thanks to exponential growth in data volume, the diversification of data sources, and the need for real-time analytics, complexities are intensifying.
Automation a Must for Travel Industry Data Pipelines
As is the case in other industries, travel industry businesses do not have the time or resources to manually cobble together data pipelines for every use case and every application as each emerges.
Ensuring Good Data Quality with Automated Data Pipelines
Automated data pipelines address or completely eliminate most of the common factors that can impact data quality.
Transforming Data Engineering with Generative AI
Data engineers can use generative AI in multiple ways in their jobs. Some key use cases include using the technology to prep and clean data, write code, and more.
The Case for Automated ETL Pipelines
Automated ETL paves the way for more accurate insights, informed decision-making, and a nimble response to the fluid nature of data sources and structures.
End-to-End Data Pipelines: Hitting Home Runs in Data Strategy
End-to-end data pipelines serve as the backbone for organizations aiming to harness the full potential of their data.
Top Data Pipeline Challenges and What Companies Need to Fix Them
Companies need robust, fault-tolerant pipelines with proper error handling, monitoring, and alerting mechanisms. Many are turning to innovative technologies like generative AI to help.
Data Automation Engineer: Skills, Workflow, and Business Impact
In recent years, the data space has transformed significantly. Advanced tools and platforms are now taking away the cumbersome tasks of managing outdated architectures and intricate codebases.
MLOps Automation Key To Accelerating Machine Learning Projects
More organizations are coming to the realization that MLOps can accelerate development and maintain consistency throughout an ML projects lifecycle.
Startup Data Team Spotlight: Why Brazen Chose Build over Buy
In the build vs buy paradigm, the decision ultimately rests on understanding your startup’s unique constraints and needs. As Brazen’s experience shows, buying from a trusted vendor like Ascend can empower startups to overcome both organizational and technical constraints.
5 Best Practices for Data Pipelines
As businesses become more data-orientated, one of the most common issues run into is the constant rise in the volume and number of sources of data, which is often not properly treated and leads to siloed data.
Supercharging Business Innovation with Automated Data Pipelines
In an ever-evolving global economy, businesses are in a continuous race to harness data as a powerful propellant for innovation. Despite this, prompt and efficient data delivery remains a formidable challenge.
Report Documents the Cost Benefits of Automated Data Pipelines
The need to provide access to data for reporting, analytics, and other business purposes is constantly growing. So, too, is the complexity of setting up and maintaining data pipelines that are needed to deliver that data to the right data consumer at the right time.
The Post-Modern Data Stack: Boosting Productivity and Value
The “modern data stack” has become increasingly prominent in recent years, promising a streamlined approach to data processing. However, this well-intentioned foundation has begun to crack under its own complexity.
Why Now Is the Time to Automate Data Pipelines
For decades, business operations involving data were relatively straightforward. Organizations extracted structured and relational data maintained in enterprise systems and performed their data analytics, reporting, and querying on that data.
How to Ensure Data Integrity at Scale By Harnessing Data Pipelines
Right now, at this moment, are you prepared to act on your company’s data? If not, why? At Ascend, we aim to make the abstract, actionable. So when we talk about making data usable, we’re having a conversation about data integrity.
Why the Manual Creation of Data Pipelines Must Give Way to Advanced Trends
Organizations that embrace the shift away from manual pipeline creation will position themselves to harness the full potential of their data assets and stay ahead in their data-driven journeys.
Data Pipeline Trends in Healthcare
State health departments are under a significant amount of pressure to ensure that individual records and other health data is up-to-date and consumable for a wide variety of use cases.
Data Pipeline Optimization: How to Reduce Costs with Ascend
The costs of developing and running data pipelines are coming under increasing scrutiny because the bills for infrastructure and data engineering talent are piling up.
Data Mesh Implementation: Your Blueprint for a Successful Launch
Ready or not, data mesh is fast becoming an indispensable part of the data landscape.
Automated Data Pipelines Make it Easier to Use More Data Sources
Multiple studies over the years have documented the rise in the use of additional data sources in decision-making processes.
MLOps vs DataOps: Will They Eventually Merge?
Abbreviations for IT operations are all the rage at the moment, with DevOps kickstarting a trend of sub-methodologies of software development and operations, including DataOps, MLOps, and AIOps.
National Labs Provide a Peek into the Future of Data Sharing
Data access and data sharing are critical to businesses today. Increasingly, automated data pipelines are playing a key role.
Why the Need for the Post-Modern Data Stack?
The modern data stack is quite complex. Developers spend great amounts of time trying to get multiple tools to work together when building data pipelines.
Data Sharing Key To Smart City Project Success
Cities embarking on digital transformations, in the form of sensor network deployment and the digitization of services.
Challenges Data Pipeline Engineers Need To Overcome
With the amount of data the average organization ingests on a daily basis increasing every year, the old ways of collecting, storing, and analyzing said data are not workable in a modern, real-time environment.
Dialing Down the Dollars: Quantify and Control Your Data Costs
Creating business value from the onslaught of data can feel like captaining a high-tech vessel through uncharted waters. Data teams across business areas are cranking out data sets in response to impatient business requests.
3 Ways to Improve Data Team Productivity in the Age of Complexity
Volume, variety, and velocity of data have exponentially increased, and now every company is potentially a data company. Organizations strive to leverage data for competitive advantage.
What’s Changing Faster? Data Pipeline Tech or the Role of the Data Scientist?
The days of manual data pipeline creation are fading fast if not already long gone. The modern data stack is too complex, and the volume of pipelines needed in most businesses makes data pipeline automation a must.
Data Pipeline Basics: From Raw Data to Actionable Insights
Data pipelines are the under-the-hood infrastructure that enables modern organizations to collect, consolidate, and leverage data at scale.
Data Pipeline Automation Needs To Come Before MLOps Automation
A majority of artificial intelligence projects are expected to shift from pilot to operational in the next two years, as organizations look to put their multi-year investments into AI to work.
Data Pipeline vs ETL: Which Delivers More Value?
In the modern world of data engineering, two concepts often find themselves in a semantic tug-of-war: data pipeline vs ETL. In the early stages of data management evolution, ETL processes offered a substantial leap forward in how we handled data.
High-Performance Data Pipelines for Real-Time Decision-Making
It may seem like everyone has their AI models up and running. Announcements have appeared all over the place for companies deploying something they’re calling AI into general operations, customer experience tools, and platforms.
How Automated Data Pipeline Tools Can Help Speed To Market
Improving speed to market is critical for businesses to maintain a competitive edge and improve revenue generation.
The Hidden Challenges of the Modern Data Stack
The “modern data stack” is supposed to be like assembling a squad of data software superheroes. You’ve got one solution that’s excellent for ingestion.
The Evolving Landscape of Data Pipeline Technologies
Organizations continue to grapple with large data volumes demanding meticulous collection, processing, and analysis to glean insights. Unfortunately, many of these efforts are still missing the mark.
Data Pipeline Tools Market Size To Reach $19 Billion by 2028
Data pipeline tools are becoming more of a necessity for businesses utilizing analytics platforms, as a way to speed up the process.
Five Data Pipeline Best Practices to Follow in 2023
Data pipelines are having a moment — at least, that is, within the data world. That’s because as more and more businesses are adopting a data-driven mindset, the movement of data into and within organizations has never been a bigger priority.
What Are Intelligent Data Pipelines?
Data teams worldwide are building data pipelines with the point solutions that make up the “modern data stack.” But this approach is quite limited, and does not actually provide any true automation.
Data Pipeline Pitfalls: Unraveling the Technical Debt Tangle
Technical debt in the context of data pipelines refers to the compromises and shortcuts developers may take when building, managing, and maintaining the pipelines.
Speed To Market Issues Lead Big Data-as-a-Service Market Growth
Big data as a service is expected to see major growth in market size over the next decade, fueled by organizations automating data analytics.
The Technology Behind and Benefits of Data Pipeline Automation
A chat with Sean Knapp, founder and CEO of Ascend.io, about the challenges businesses face with data pipelines and how data pipeline automation can help.
DataOps’ Role in a Modern Data Pipeline Strategy
Increasingly, businesses are using DataOps principles to guide their data pipeline strategies, construction, and operations.
The Business Value of Intelligent Data Pipelines
Intelligent data pipelines serve as a transformative solution for organizations seeking to stay competitive in an increasingly data-driven world.
DataOps Trends To Watch in 2023
DataOps is becoming critical for organizations to ensure that data is being used in an efficient and compliant way.